Abstract
A combined model was developed using contrast-enhanced CT-based radiomics features and clinical characteristics to predict liver fibrosis stages in patients with chronic liver disease (CLD). We retrospectively analyzed multiphase CT scans and biopsy-confirmed liver fibrosis. 160 CLD patients were randomly divided into 7:3 training/validation ratio. Clinical laboratory indicators associated with liver fibrosis were identified using Spearman's correlation and multivariate logistic regression correlation. Radiomic features were extracted after segmenting the entire liver from multiphase CT images. Feature dimensionality reduction was performed using RF-RFE, LASSO, and mRMR methods. Six radiomics-based models were developed in the training cohort of 112 patients. Internal validation was conducted on 48 randomly assigned patients. Receptor Operating Characteristic (ROC) curves and confusion matrices were constructed to evaluate model performance. The radiomics model exhibited robust performance, with AUC values of 0.810 to 1.000 for significant fibrosis, advanced fibrosis, and cirrhosis. The integrated clinical-radiomics model had superior diagnostic efficacy in the validation cohort, with AUC values of 0.836 to 0.997. Moreover, these models outperformed established biomarkers such as the aspartate aminotransferase to platelet ratio index (APRI) and the fibrosis 4 score (FIB-4), as well as the gamma glutamyl transpeptidase to platelet ratio (GPR), in predicting the fibrotic stages. The clinical-radiomics model holds considerable promise as a non-invasive diagnostic tool for the assessment and staging of liver fibrosis in the patients with CLD, potentially leading to better patient management and outcomes.
Similar content being viewed by others
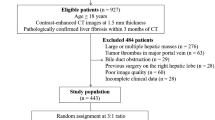
Explore related subjects
Discover the latest articles, news and stories from top researchers in related subjects.Introduction
Global public health is significantly impacted by chronic liver disease (CLD). Liver fibrosis may progress to cirrhosis, complications related to portal hypertension, and even hepatocellular cancer, which is closely linked to high morbidity and mortality in CLD1. Therefore, it is crucial to diagnose liver cirrhosis and quantify liver fibrosis as early as possible in the management of chronic liver disease2. The gold standard for staging liver fibrosis is liver biopsy. However, it carries the risk of procedure-related complications, such as severe bleeding, organ damage, pain, and death, and has limitations related to sampling error, inter-observer variability, semi-quantitative results, and high cost3.
In clinical practice, the extent of liver fibrosis is commonly evaluated using serum biomarkers. Serum markers, including aspartate aminotransferase to platelet ratio index (APRI) and the fibrosis 4 score (FIB-4), as well as the gamma glutamyl transpeptidase to platelet ratio (GPR) rely on clinical and biochemical indicators including serum aspartate aminotransferase (AST), alanine aminotransferase (ALT) levels, and serve as viable alternatives to liver biopsy for monitoring changes in liver fibrosis caused by various factors4. However, there are also limitations to these metrics, since the biomarkers do not target a particular organ and their measurement is highly dependent on how quickly they are excreted and cleared5. A number of imaging-based methods have been investigated to evaluate the extent of liver fibrosis6,7,8,9. Magnetic resonance imaging (MRI) and ultrasonography (US) elastography have been the most successful methods for measuring liver stiffness in clinical practice7,8. Nevertheless, inaccuracies in measuring deep liver tissues, and their usability and clinical relevance are compromised by the fact that ascites or severe obesity can significantly affect their performance. The 2018 American Association for the Study of Liver Diseases(AASLD) Practice Guidelines suggest using multi-phase CT or MRI for initial diagnosis in high-risk patients with abnormal monitoring results10. Enhanced CT offers more information than plain CT scans and has advantages over MRI, including lower cost, fewer contraindications, widespread availability, and excellent whole-organ imaging capabilities11. However, CT enhancement has drawbacks like radiation exposure and potential iodine allergies.
Radiomics can turn images into extensive quantitative data, enabling the extraction of additional biological information12, As a clinical decision-making tool in liver diseases, radiomics analysis primarily aims to detect hepatocellular carcinoma13, diagnose indeterminate hepatic nodules14, and assess the risk of recurrence of hepatocellular carcinoma15. The utilization of radiomics for identification of distinct presentations of liver disease, like clinically significant portal hypertension16 and hepatic fibrosis staging17,18,19, has not been extensively investigated in previous studies. It remains uncertain which phase, arterial, venous, or delayed, has the potential to improve the accuracy of liver fibrosis staging analysis.
The objective of this study was to investigate whether adding routine clinical biomarkers to radiomics signatures improves the accuracy of liver fibrosis staging using CT-based radiomics data. The secondary aim was to compare the accuracy of the clinical-radiomic model and specific clinical indicators in diagnosing liver fibrosis severity.
Materials and methods
The Institutional Review Board (IRB) at the Affiliated Hospital of Guizhou Medical University. approved this retrospective study. The study adhered to ethical guidelines. Patient confidentiality and data protection measures were strictly followed to ensure privacy and anonymity.
Patients
The dataset comprises multi-phase abdominal CT enhanced imaging and liver histopathological examination data from 110 patients who were treated at our hospital between 2017 and 2022.
Inclusion criteria were: (1) Patients with chronic liver disease. (2) Multiphase contrast agent-enhanced CT examinations. (3) Pathologic findings within 1 month of CT examination. (4) No previous liver surgery or evidence of malignant tumors. Exclusion criteria were: (1) Lack of enhanced CT images. (2) Incomplete clinical data, such as incomplete laboratory data or histologic staging. (3) Poor image quality preventing analysis, such as heavy image artifacts. (4) The focal liver lesions detected by CT images (maximum diameter > 5 cm). (5) History of previous liver surgery. Following these criteria, 826 cases of chronic liver disease were initially collected, with 716 patients excluded, leaving a final inclusion of 110 eligible patients (Fig. 1).
Additionally, the dataset includes CT-enhanced imaging data from 50 patients diagnosed with cirrhosis. The enhanced images of these 50 cases of decompensated cirrhosis, which are characterized by wavy margins of the liver, portal hypertension, splenomegaly, and ascites, were selected by two radiologists with 8 years of clinical experience in abdominal radiology (Fig. 1).
Pathological stages of liver fibrosis
110 patients with CLD had confirmed histological results by pathological biopsy. The liver samples were examined by an experienced pathologists who was blinded to the clinical and laboratory data, using the Scheuer scoring system20. The stages of liver fibrosis were as follows: S0(no fibrosis), S1(mild fibrosis, no septum), S2 (moderate fibrosis, few intervals), S3(severe fibrosis, many intervals, no cirrhosis), S4 (cirrhosis). According to this study, pathological stage ≥ S2 and ≥ S3 were used to define significant fibrosis and advanced fibrosis, respectively.
50 patients of enhanced images from cirrhotic patients were selected by two radiologists and classified as stage S4 (cirrhosis).
CT image acquisition
The patient's abdominal enhanced digital medical images were retrieved from the Picture Archiving and Communication System (PACS) of the Affiliated Hospital of Guizhou Medical University.
The patients primarily underwent imaging using 128-slice MSCT scanner (SOMATOM Definition AS + , Siemens, Germany). The scanning range covered the upper abdomen.
Radiomic feature extraction and selection
Enhanced 1 mm DICOM images of 160 patients were converted to neuroimaging informatics technology initiative (NIfTI) image and automatically segmented using 3D-Unet liver segmentation model. Manual modification after the liver segmentation was performed using 3D Slicer (V5.3.0, https:// www.slicer.org/). Pyradiomics was used to extract radiomic texture features from enhanced images of 160 patients. Feature stability was assessed by randomly selecting 40 patients and performing segmentation twice, with intra-class correlation coefficient (ICC) used to retain only features with values greater than 0.8 for subsequent analysis. Z-scores normalized stable radiomic features values. Next, We employed the Spearman correlation coefficient (p < 0.05) to assess the correlation between stable radiomic features and fibrosis staging. To address the high number of features, we used random forest recursive feature elimination (RF-RFE) to select the top 50 features, and least absolute shrinkage and selection operator (LASSO) to reduce the feature. If the features still exceed 10 features, we employed the minimum redundancy maximum relevance (mRMR) method to select the most representative features count to 10 or below for support vector classifier (SVC) modeling. The parameters of the SVC model were determined through grid search, which was manually tuned to achieve the best performance and obtain the most optimal results. The training set underwent 10 repetitions of random 5-fold cross-validation, this process involves training data and testing data. The penalty parameter was fine-tuned during this process. The same method is used to process images in the arterial phase, venous phase, and delayed phase. The final model evaluation involved the use of area under the curve (AUC) and confusion matrix.
The radiomics quality score (Radscore) is calculated from the linear combination of features selected by LASSO and the weighting coefficients corresponding to each radiomic feature. The calculation formula for Radscore is as follows:
where β1-βi represent the coefficients of the variables selected through LASSO analysis, and X1-Xi represent the corresponding values of these variables. (Fig. 2) shows a concise flow diagram that clarifies the entire study.
The radiomic workflow. The procedure encompassing tasks such as ROI segmentation, features extraction, features selection, clinical application and analysis. (a) Achieving automatic segmentation of the entire liver ROI on enhanced CT images using 3D-unet. followed by manual refinement for the entire liver parenchyma delineation. (b) Extracted radiomic features, including first-order statistics, textural features, and wavelet-based. transformations. (c) Models were developed using SVC, including the Radscorearterial/venous/delayed model and the Clinical-Radiomicsarterial/venous/delay model. The performance of the established models was evaluated using ROC curves, DCA curves, and confusion matrices. ROC, receiver operator characteristic, DCA, decision curve analysis.
Clinical factors selection
In the selection of clinical factors, we implemented a three-step process. First, preliminary screening of factors with significant correlations was carried out using Spearman correlation analysis (P < 0.05). Second, multivariate logistic regressions with backward stepwise selection was applied to choose the factors for predicting significant fibrosis stage. Third, the variance inflation factor (VIF) is used to exclude collinearity among variables, where VIF greater than 10 will be excluded21. The APRI, FIB-4, and GRP were determined through the application of the following formulas:22,23,24
In the formula (2),(3),(4), ULN represents the upper limit of the normal value, and PLT refers to the platelet count, expressed in terms of platelets per liter (10^9). An integrated SVC model was created by combining the radiomics signature with clinical biomarkers.
Statistical analysis
Mean ± SD represented continuous variables analyzed through Kruskal–Wallis rank test. Categorical variables were represented as proportions and analyzed by Chi-squared or Fisher's exact test. Reliability was assessed using the ICC, with consistency divided into poor (< 0.5), medium (0.51—0.80), good (0.81—0.89) or excellent (≥ 0.9). The nomogram model was constructed based on the Radscore and clinical risk factors. The AUC was employed to assess the predictive accuracy of the models. The threshold value is determined by the F1-score index, which is an ideal combination of sensitivity and specificity. The corresponding sensitivity, specificity and accuracy were estimated. The prediction performance was further assessed by decision curve analysis (DCA) to determine its clinical utility. A two-sided p < 0.05 was considered statistically significant. All statistical analyses were conducted in R software (version 4.3.0, https://cran.r-project.org/src/base/R-4/) and Python (version 3.8.2, https://www.python.org/downloads/release/python-382/).
Results
Study population
We acquired comprehensive demographic and clinical information about the patients (Table 1). Pathological staging of liver fibrosis (S0-S4) corresponds to arterial, venous, and contrast-enhanced CT images as presented in (Fig. 3).
The changes in the arterial, venous, and delayed phases of enhanced CT images from S0 to S4. In S01, the liver appears normal on imaging. In S2-4, the portal vein widens and the liver-spleen volume ratio decreases. By S4, the liver edge exhibits a wavy pattern of changes, and the liver fissures become wider. Multiple tortuous small blood vessels are observed in the gastric fundus, along with ascites.
Identification of predictive clinical factors
Routine clinical factors including Creatine Kinase-MB (CKMB), Hemoglobin (HB), AST, Alkaline phosphatase (ALP), Gamma-glutamyl transferase (GGT), Total bile acid (TBA), Total bilirubin (TBIL), Direct bilirubin (DBiL), Urea (UR), Red blood Cell (RBC), C-reactive protein (CRP), Albumin-globulin ratio (A/G), High-density lipoprotein cholesterol (HDL-C), Low-density lipoprotein cholesterol (LDL-C), Total Cholesterol (TC), Lipoprotein (LP), Myoglobin (MYO) and Pro-B-type natriuretic peptide (PBNP) were also related to significant fibrosis (P < 0.05 for all). A multivariable logistic regression analysis identified PLT, ALP, A/G, UR, LDL-C, and TC as independent predictors for significant fibrosis (Table 2). We have developed a clinical model based on the comprehensive analysis of PLT, ALP, A/G, UR, LDL-C, and TC laboratory indicators. The AUC results of the clinical model are 0.811, 0.850, and 0.953 for significant fibrosis, advanced fibrosis, and cirrhosis (Table 3).
Identification of predictive FIB-4 APRI GPR
The analysis encompassed the selection of specific clinical biomarkers, namely APRI, FIB-4, and GPR. The AUC of the APRI model is 0.757 in the diagnosis of significant fibrosis, while the AUCs were 0.796 and 0.821 in the diagnosis of advanced fibrosis and cirrhosis, respectively. The AUC results of FIB-4 were 0.801, 0.880 and 0.931, respectively. The AUC results of GPR were 0.750, 0.746 and 0.758, respectively (Table 3). We employed DeLong test statistics to compare the performance of different models. The Clinical-Radiomicsarterial model demonstrates robust predictive abilities in disease prognostication. Furthermore, the Radscorearterial, APRI, GPR, and FIB-4 models exhibited varying degrees of predictive abilities (Table 4).
Feature reduction and establishment of radiomics model
1218 features were extracted from arterial phase, venous phase and delayed phase respectively, resulting in a total of 3654 features. Select stable features with high reproducibility through ICC for further analysis (684 arterial phase features, 645 venous phase features, 496 delayed phase features).
In the arterial phase, the LASSO and mRMR method identified a set of 10 features, including 3 first-order statistics, 6 texture features, and 1 wavelet-based transformations features. In the venous phase, 6 features were selected, consisting of 4 first-order statistics features and 2 texture features. In the delayed phase, 6 features were selected, comprising of 3 first-order statistics features and 3 texture features. These features were trained to staging fibrosis. The models was constructed using the SVC algorithm. All models were trained and validated using the parameters C = 0.5, gamma = scale, kernel = rbf, probability = True, and with random state = 20.
As for the validation cohort, the radiomics models based on multi-phase CT images showed the performance with AUCs of 0.839 (95% CI 0.835–0.844), 0.816 (95% CI 0.813–0.818), 0.870 (95% CI 0.869- 0.871) for the arterial phase; 0.816 (95% CI 0.812- 0.820), 0.884 (95% CI 0.882–0.886), 1.000 (95% CI 1.000- 1.000) for the venous phase; 0.810 (95% CI:= 0.806- 0.814), 0.882 (95% CI 0.880- 0.884), 0.998 (95% CI 0.998- 0.998) for the delay phase in the diagnosis of significant fibrosis (S01 vs. S234), advanced fibrosis (S012 vs. S34), and cirrhosis (S0123 vs. S4), respectively (Table 3).
The construction nomograms and decision curve analysis for Clinical-Radiomics
We developed the Clinical-Radiomicsarterial nomogram (Fig. 4) combines the Radscorearterial with six clinical markers (PLT, ALP, A / G, UR, LDL-C, and TC). In the validation cohort, the AUCs for combination model of Clinical-Radiomicsarterial were 0.854 (95% CI 0.851- 0.857), 0.863 (95% CI 0.861- 0.866), 0.960 (95% CI 0.958- 0.961) for differentiating significant fibrosis, advanced fibrosis, and cirrhosis, respectively; The AUCs of Clinical-Radiomicsvenous were 0.836 (95% CI 0.833- 0.838), 0.862 (95% CI 0.860- 0.864), and 0.997 (95% CI 0.996- 0.997); The AUCs of Clinical-Radiomicsdelay were 0.838 (95% CI 0.835- 0.841), 0.863 (95% CI 0.862- 0.865), 0.996 (95% CI 0.996- 0.997). The performance of the validation set was demonstrated in (Table 3).
The nomograms of the Clinical-Radiomicsarterial model for evaluating fibrosis stage. Nomograms were constructed to evaluate significant fibrosis, advanced liver fibrosis and cirrhosis, respectively. Each variable specifies a point on the top axis by drawing a line upwards. The sum of these numbers is located on the “total points” axis, and a straight line is drawn down on the “probability” axis to calculate the likelihood of staging liver fibrosis.
The diagnostic performance of the Clinical-Radiomicsarterial model was outstanding in both the training and validation sets, warranting attention, with AUC values ranging from 0.833 to 0.923 and 0.854 to 0.960, with accuracy of 80.64–91.07% and 76.62–87.04% for staging fibrosis. The performance of the Clinical-Radiomics model was assessed using ROC analysis, as depicted in (Fig. 5). The performance of the Clinical-Radiomicsarterial model was evaluated using a matrix, as illustrated in (Fig. 6). Besides, we further evaluated the Clinical-Radiomicsarterial model in predicting liver fibrosis by DCA (Fig. 7). The DCA has shown that employing a Clinical-Radiomics nomogram to predict fibrosis status provides more benefit than treating all or none of the patients.
Discussion
The results of this study clearly demonstrate that the radiomics model is highly effective in distinguishing between various stages of liver fibrosis, exhibiting AUC values ranging from 0.810 to 1.000. The integration of clinical parameters with radiomics data further enhances diagnostic accuracy, as indicated by AUC values between 0.836 and 0.997 in the validation cohort, and achieves a commendable accuracy of 71.42 to 96.54% in staging hepatic fibrosis. Notably, these models have also been shown to significantly outperform traditional non-invasive biomarkers such as GPR, APRI, and FIB-4. The results indicate that the clinical-radiomics model manifests substantial potential as a sophisticated non-invasive diagnostic modality for the nuanced assessment and accurate staging of hepatic fibrosis, presaging enhanced patient management and prognostic outcomes.
Previous study has suggested that elastography methods entails a higher cost compared to alternative noninvasive indicators25, and CT-based radiomics has the potential to identify subtle alterations throughout the progressive stages of liver fibrosis26, However, the study only included the extraction of radiomic features from 2D images at the portal vein level of liver, and did not compare the performance of arterial, venous, and delayed phase models based on multiphase CT images in radiomic models. In comparison, our study employed the trained 3D-UNet liver segmentation software for the automatic segmentation of the entire liver. Subsequently, we conducted a comparative analysis of multiphase CT images, encompassing the arterial phase, venous phase, and delayed phase. Hu et al. presented that venous phase CT images were chosen to develop and validate a CT-based radiomics nomogram for distinguishing between non-advanced and advanced liver fibrosis27. Our research findings indicate that the model based on the Radscorearterial shows a higher AUC in the significant fibrosis (S01vs.S234) group. Meanwhile, in the advanced fibrosis (S012vs.S34) and cirrhosis (S0123vs.S4) groups, the AUC of the the Radscorevenous/delayed model was higher.
Prior research have unveiled that FIB-4 excels in accurately identifying cirrhosis (F4) while displaying suboptimal performance in ruling out significant fibrosis (≥ F2) , APRI exhibits solely moderate sensitivity and accuracy in appraising fibrosis connected to HBV28. Previous studies also have demonstrated GPR is an inexpensive, simple, and easy-to-calculate model for anticipating the extent of liver fibrosis in patients with chronic hepatitis B29. According to our results, the three specific clinical indicators were effective in determining the stage of liver fibrosis with the AUCs of 0.750–0.931 and accuracy of 57.29–88.00% in the validation set. However, the diagnostic efficacy is lower compared to our clinical-radiomics model.
Several constraints in this study should be noted. First, due to its retrospective nature, inherent selection biases are unavoidable. A future prospective, multicenter study addressing the same research question could offer significant improvements. Second, the limited sample size of patients with F3 liver fibrosis in the current study poses a potential constraint, making machine learning models susceptible to the bias-variance tradeoff. Third, significant disparities in sample sizes for liver fibrosis across different etiologies hinder the realization of stratified research objectives. Finally, variations in CT scanners may impact the study's outcome.
Conclusion
We developed a combined prediction model utilizing multiphase CT-based radiomics features and clinical biomarker, and the combined model exhibited strong performance in predicting fibrosis stage with CLD patients. The clinical-radiomics nomogram has the potential to assist in clinical decision-making, offering potential benefits in the patients with CLD.
Data availability
The datasets generated during and/or analyzed during the current study are available from the corresponding author on reasonable request.
Abbreviations
- A/G:
-
Albumin-globulin ratio
- ALB:
-
Albumin
- ALP:
-
Alkaline phosphatase
- ALT:
-
Alanine aminotransferase
- APRI:
-
Aspartate aminotransferase to platelet ratio index
- AST:
-
Aspartate aminotransferase
- AUC:
-
Area under the curve
- HBV:
-
Hepatitis B virus
- CKMB:
-
Creatine kinase-MB
- CLD:
-
Chronic liver disease
- CRP:
-
C-reactive protein
- CT:
-
Compute tomography
- cTn:
-
Cardiac troponin
- DBiL:
-
Direct bilirubin
- DCA:
-
Decision curve analysis
- FIB-4:
-
Fibrosis 4 score
- FOV:
-
Field of view
- GGT:
-
Gamma-glutamyl transferase
- GLB:
-
Globulin
- GPR:
-
Gamma glutamyl transpeptidase to platelet ratio
- HB:
-
Hemoglobin
- HDL-C:
-
High-density lipoprotein cholesterol
- ICC:
-
Intra-class correlation coefficient
- IRB:
-
Institutional review board
- LASSO:
-
Least absolute shrinkage and selection operator
- LDL-C:
-
Low-density lipoprotein cholesterol
- LP:
-
Lipoprotein
- MRI:
-
Magnetic resonance imaging
- mRMR:
-
Minimum redundancy maximum relevance
- MSCT:
-
Multi-slice computed tomography
- MYO:
-
Myoglobin
- PBNP:
-
Pro-B-type natriuretic peptide
- PLT:
-
Platelet
- Radscore:
-
Radiomics quality score
- RBC:
-
Red blood cell
- RF-RFE:
-
Random forest recursive feature elimination
- ROC:
-
Receiver operating characteristic curve
- TBA:
-
Total bile acid
- TBIL:
-
Total bilirubin
- TC:
-
Total cholesterol
- TP:
-
Total protein
- UR:
-
Urea
- US:
-
Ultrasonography
- VIF:
-
Variance inflation factor
- WBC:
-
White blood cell
References
Cheemerla, S. & Balakrishnan, M. Global epidemiology of chronic liver disease. Clin. Liver Dis. (Hoboken) 17(5), 365–370 (2021).
Castera, L. Invasive and non-invasive methods for the assessment of fibrosis and disease progression in chronic liver disease. Best Pract. Res. Clin. Gastroenterol. 25(2), 291–303 (2011).
Bravo, A. A., Sheth, S. G. & Chopra, S. Liver biopsy. N. Engl. J. Med. 344(7), 495–500 (2001).
Loomba, R. & Adams, L. A. Advances in non-invasive assessment of hepatic fibrosis. Gut 69(7), 1343–1352 (2020).
Grigorescu, M. Noninvasive biochemical markers of liver fibrosis. J. Gastrointest. Liver. Dis. 15(2), 149–159 (2006).
Lubner, M. G., Malecki, K., Kloke, J., Ganeshan, B. & Pickhardt, P. J. Texture analysis of the liver at MDCT for assessing hepatic fibrosis. Abdom. Radiol. (NY). 42(8), 2069–2078 (2017).
Herrmann, E. et al. Assessment of biopsy-proven liver fibrosis by two-dimensional shear wave elastography: An individual patient data-based meta-analysis. Hepatology. 67(1), 260–272 (2018).
Singh, S. et al. Diagnostic performance of magnetic resonance elastography in staging liver fibrosis: A systematic review and meta-analysis of individual participant data. Clin. Gastroenterol. Hepatol. 13(3), 440-451.e6 (2015).
Xiao, G. et al. Comparison of laboratory tests, ultrasound, or magnetic resonance elastography to detect fibrosis in patients with nonalcoholic fatty liver disease: A meta-analysis. Hepatology 66(5), 1486–1501 (2017).
Marrero, J. A. et al. Diagnosis, staging, and management of hepatocellular carcinoma: 2018 practice guidance by the American association for the study of liver diseases. Hepatology 68(2), 723–750 (2018).
Wang, J. et al. Radiomics analysis of contrast-enhanced CT for staging liver fibrosis: An update for image biomarker. Hepatol. Int. 16(3), 627–639 (2022).
Gillies, R. J., Kinahan, P. E. & Hricak, H. Radiomics: Images are more than pictures. Data. Radiol. 278(2), 563–577 (2016).
Wei, J. et al. Radiomics in liver diseases: Current progress and future opportunities. Liver Int. 40(9), 2050–2063 (2020).
Mokrane, F. Z. et al. Radiomics machine-learning signature for diagnosis of hepatocellular carcinoma in cirrhotic patients with indeterminate liver nodules. Eur. Radiol. 30(1), 558–570 (2020).
Ji, G. W. et al. Machine-learning analysis of contrast-enhanced CT radiomics predicts recurrence of hepatocellular carcinoma after resection: A multi-institutional study. EBioMedicine https://doi.org/10.1016/j.ebiom.2019.10.057 (2019).
Liu, F. et al. Development and validation of a radiomics signature for clinically significant portal hypertension in cirrhosis (CHESS1701): A prospective multicenter study. EBioMedicine https://doi.org/10.1016/j.ebiom.2018.09.023 (2018).
Park, H. J. et al. Radiomics analysis of gadoxetic acid-enhanced MRI for staging liver fibrosis. Radiology. 290(2), 380–387 (2019).
Zhou, Z. et al. Liver fibrosis assessment using radiomics of ultrasound homodyned-k imaging based on the artificial neural network estimator. Ultrason. Imaging. 44(5–6), 229–241 (2022).
Zhang, D. et al. Radiomics nomograms based on R2* mapping and clinical biomarkers for staging of liver fibrosis in patients with chronic hepatitis B: A single-center retrospective study. Eur. Radiol. 33(3), 1653–1667 (2023).
Scheuer, P. J. Classification of chronic viral hepatitis: A need for reassessment. J. Hepatol. 13(3), 372–374 (1991).
O’brien, R. M. a caution regarding rules of thumb for variance inflation factors. Quality Quantity https://doi.org/10.1007/s11135-006-9018-6 (2007).
Wai, C. T. et al. A simple noninvasive index can predict both significant fibrosis and cirrhosis in patients with chronic hepatitis C. HEPATOLOGY 38(2), 518–526 (2010).
Vallet-Pichard, A. et al. FIB-4: an inexpensive and accurate marker of fibrosis in HCV infection comparison with liver biopsy and fibrotest. Hepatology https://doi.org/10.1002/hep.21669 (2007).
Lemoine, M. et al. The gamma-glutamyl transpeptidase to platelet ratio (GPR) predicts significant liver fibrosis and cirrhosis in patients with chronic HBV infection in West Africa. Gut 65(8), 1369–1376 (2016).
EASL-ALEH Clinical Practice Guidelines. Non-invasive tests for evaluation of liver disease severity and prognosis. J. Hepatol. 63(1), 237–264 (2015).
Wang, J. C. et al. A radiomics-based model on non-contrast CT for predicting cirrhosis: Make the most of image data. Biomark Res. https://doi.org/10.1186/s40364-020-00219-y (2020).
Hu, P. et al. A combination model of radiomics features and clinical biomarkers as a nomogram to differentiate nonadvanced from advanced liver fibrosis: A retrospective study. Acad. Radiol. https://doi.org/10.1016/j.acra.2020.08.029 (2021).
Zeng, D. W., Dong, J., Liu, Y. R., Jiang, J. J. & Zhu, Y. Y. Noninvasive models for assessment of liver fibrosis in patients with chronic hepatitis B virus infection. World J. Gastroenterol. 22(29), 6663–6672 (2016).
Purkayastha, S., Jha, A. K., Kumar, R., Dayal, V. M. & Jha, S. K. Serum gamma-glutamyl transpeptidase-to-platelet ratio as a noninvasive marker of liver fibrosis in chronic hepatitis B. Cureus. 15(1), e33744 (2023).
Acknowledgements
The authors greatfully thank all the participants at Guizhou Medical University; we are also thankful to StudyForBetter Team who contributed their best research spirits to this work.
Funding
Funding for the Excellent Reserve Talents in the Discipline of Affiliated Hospital of Guizhou Medical University,gyfyxkyc-2023-13,Guizhou Provincial Science and Technology Projects,Qiankehejichu-ZK[2023]353,Cultivation Project of National Natural Science Foundation of China by The Affiliated of Guizhou Medical University,gyfynsfc[2023] -03,Guizhou Province High-level Innovative Talent Program,gzwijrs 2023-019.
Author information
Authors and Affiliations
Contributions
Writing—original draft, Methodology, Data curation, Formal analysis,Validation:[Maowen Tang ], Writing—original draft, Methodology, Data curation,Visualization, Software. [Yuhui Wu ], Writing—review &editing, Methodology, Data curation, Investigation. [Na Hu], Data curation, Writing—review &editing, Formal analysis:[Chong Lin], Data curation, Writing—review &editing:[Jian He], Writing—review &editing, Methodology:[Xing Xia, Meihua Yang], Writing—review &editing, Supervision, Formal analysis, Conceptualization, Funding acquisition:[Pinggui Lei, Peng Luo]. All authors reviewed the manuscript.
Corresponding authors
Ethics declarations
Competing interests
The authors declare no competing interests.
Additional information
Publisher's note
Springer Nature remains neutral with regard to jurisdictional claims in published maps and institutional affiliations.
Rights and permissions
Open Access This article is licensed under a Creative Commons Attribution-NonCommercial-NoDerivatives 4.0 International License, which permits any non-commercial use, sharing, distribution and reproduction in any medium or format, as long as you give appropriate credit to the original author(s) and the source, provide a link to the Creative Commons licence, and indicate if you modified the licensed material. You do not have permission under this licence to share adapted material derived from this article or parts of it. The images or other third party material in this article are included in the article’s Creative Commons licence, unless indicated otherwise in a credit line to the material. If material is not included in the article’s Creative Commons licence and your intended use is not permitted by statutory regulation or exceeds the permitted use, you will need to obtain permission directly from the copyright holder. To view a copy of this licence, visit http://creativecommons.org/licenses/by-nc-nd/4.0/.
About this article
Cite this article
Tang, M., Wu, Y., Hu, N. et al. A combination model of CT-based radiomics and clinical biomarkers for staging liver fibrosis in the patients with chronic liver disease. Sci Rep 14, 20230 (2024). https://doi.org/10.1038/s41598-024-70891-9
Received:
Accepted:
Published:
DOI: https://doi.org/10.1038/s41598-024-70891-9
- Springer Nature Limited