Abstract
There has been growing attention for Large Language Models and conversational agents, and their capabilities and benefits. In addition, there is a need to look at the various costs, harms, and risks involved in their development and deployment. In order to contribute to the development and deployment of ‘trustworthy AI’, we propose to organize ethical reflection and deliberation, following the seven key requirements of the European Commission’s High-Level Expert Group on AI (2019). We propose to look at these requirements through four different ethical perspectives— consequentialism, duty ethics, relational ethics, and virtue ethics; and to look at different levels of the sociotechnical system—individual, organization, and society. We present a case study of ChatGPT, to illustrate how this approach works in practice, and close with a discussion of this approach.
Similar content being viewed by others
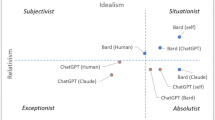
Explore related subjects
Discover the latest articles, news and stories from top researchers in related subjects.Avoid common mistakes on your manuscript.
1 Introduction
The development of Large Language Models (LLMs) has been an incremental process, but particularly the public release of ChatGPT, an LLM-based conversational agent, in November 2022, sparked a worldwide hype and even speculation about impeding Artificial General Intelligence (AGI). Articles in both popular and academic publications have discussed diverse opportunities, challenges, and implications of conversational agents (e.g., Dwivedi et al. 2023). The field is developing so fast, that there is hardly time to properly assess what is going on. For many organizations, governments, companies, and citizens, key questions are: What can it do exactly? Is it hype or real? What are the various ethical issues? It is this last question that we aim to (partially) address in this paper. Below, we will discuss several ethical issues aspects of one LLM-based conversational agent: ChatGPT.
The authors have worked in multiple applied research and innovation projects, with numerous clients and partners, on the development and evaluation of AI systems, and aiming to integrate concerns for ethical aspects in these projects. It is from this vantage point that we are interested in the ethical aspects of conversational agents. We have observed that ethical concerns often remain implicit; the people involved rarely explicitly discuss ethical perspectives and aspects. Conversely, we propose that making such perspectives and aspects more explicit, and organizing reflection and deliberation, is necessary, if we want to move ‘from principles to practices’ (Morley et al. 2020). Such ethical reflection and deliberation are urgent when AI systems are deployed in practice; especially if people’s safety and fundamental rights are at stake. In this article we discuss an approach to organize ethical reflection and deliberation, around the seven key requirements of the European Commission’s High-Level Expert Group on AI (HLEG) (2019).
There are diverse approaches to integrate ethical aspects in the development and deployment of technologies; methods can be used at the start of development, during development, or after development (Reijers et al. 2018). We propose that integrating ethical aspects during development and deployment would be most useful, especially when this is part of an iterative development process, like CRISP-DM (Martínez-Plumed et al. 2021; Shearer 2000). Furthermore, we propose to use different ethical perspectives more explicitly. Notably, we propose to use consequentialism, duty ethics, relational ethics, and virtue ethics (Van de Poel and Royakkers 2011), and to use them in parallel, as complimentary perspectives. Moreover, we understand ethics as an iterative and participatory process of ethical reflection, inquiry, and deliberation (Steen 2023a, b). The task for the people involved is then to make room for such a process and to facilitate relevant people to participate. Such a process can have three (iterative) steps:
-
Identify issues that are (potentially) at play in the project and reflect on these. A handful of issues works best (if there are more, one can cluster; if there are less, one can explore more.)
-
Organize dialogues with relevant people, both inside and outside the organization, for example, stakeholders, to inquire into these issues from diverse perspectives and to hear diverse voices.
-
Make decisions, for example, between different design options and test these in experiments; this promotes transparency and accountability. The key is to steer the project more consciously, explicitly, and carefully.
Our focus is on the first step (identify issues); below, we identify and discuss a range of ethical aspects of one specific LLM-based conversational agent: ChatGPT. The second step (organize dialogues) and the third step (make decisions) are outside the current article’s scope. Below, we will introduce the ingredients of our approach: a modest form of systems thinking; four complementary ethical perspectives; and the HLEG’s seven key requirements. Then we illustrate our approach with a case study of ChatGPT. This case study is also meant to explore how different ethical perspectives are relevant to different key requirements. We close the paper with a discussion of our approach.
2 Systems thinking
In our approach, we follow a modest form of systems thinking (Meadows 2008); we understand an AI system as part of a larger sociotechnical system and look at three levels of analysis:
-
Individual; how people can interact with a conversational agent and, for example, can benefit or suffer from that;
-
Organization; how an organization deploys a conversational agent, for example, in a service they provide;
-
Society; how, for example, the deployment of a conversational agent leads to benefits and costs for different groups or for the environment, in terms of the use of material and energy.
Our approach to understand an AI system as part a sociotechnical system and to look at different aggregation levels, is similar to the approach of Weidinger et al. (2023).Footnote 1 It is also slightly different. Weidinger et al. discuss three layers: Capability, the ‘AI systems and their technical components’ (typically ‘evaluated in isolation’); Human interaction, ‘the experience of people interacting with a given AI system’; and Systemic impact, ‘the impact of an AI system on the broader systems in which it is embedded, such as society, the economy, and the natural environment’. Our approach differs in that we propose not to study the AI system itself. We will look at an AI system always in its context (on the level of the individual, organization, and society). There are topics, however, where it makes sense to turn to ‘technical methods’ (rather than ‘non-technical methods’) (High-Level Expert Group on Artificial Intelligence 2019, p. 8); for example, in order to evaluate a technical requirement, like robustness (see below).
Furthermore, we propose to discuss the organizational level—a level of analysis that Weidinger et al. do not distinguish. We believe this level of analysis is valuable because it can enable organizations to reflect on how they practically deploy and use LLMs and conversational agents. Critically, this level is where they have agency. Moreover, we propose to look at the interactions between technology and society in terms of reciprocity, rather than in terms of ‘impact’, which incorrectly suggests a one-directional causal relationship. Building on insights from Science and Technology Studies (Oudshoorn and Pinch 2003), we acknowledge that reciprocal relationship exists between technology and society: society affects the ways in which technologies are used, and usage of technologies affects processes in society.
In various projects, we have found this systems thinking approach worthwhile: to move back and forth between these aggregation levels: to zoom-out and zoom-in. When people discuss some user interface detail, one can invite them to zoom-out and ask questions about the underlying business model and issues like fairness or inclusion. Or, conversely, if they discuss a concept like fairness in rather abstract terms, one can invite them to zoom-in and discuss how a specific user interface element can promote, or corrode, fairness in terms of accessibility or usability.
3 Ethical perspectives
In ethics of technology, it is common to use different ethical perspectives, notably: consequentialism, duty ethics (deontology), relational ethics, and virtue ethics (Van de Poel and Royakkers 2011, pp. 77–78). Moreover, in the tradition of applied ethics (Van de Poel and Royakkers 2011, pp. 105–106) we propose to combine these perspectives. This concurs with what people do in innovation projects; different people can (implicitly! ) use different ethical perspectives at different moments (Steen, Neef, Schaap 2021). They can discuss positive and negative impacts of their project’s outcomes (consequentialism), or they talk about various obligations and regulations, regarding privacy (duty ethics). And sometimes (but less often, according to our observation in projects) they talk about the impact of technology on interactions between people, in customer care (relational ethics), or they reflect on how an application can contribute to people’s abilities to live well together (virtue ethics). Mostly, however, they do that implicitly.
Our contribution is that we make these ethical perspectives more explicit. Critically, these perspectives have different assumptions and logics. One may therefore argue that, in theory, they are incompatible. In practice, however, they can very well be combined (Alfano 2016, pp. 14–18) (Steen, Neef, Schaap 2021; Steen et al. 2023); each perspective can draw attention to a different aspect of the project at hand. A key advantage of this side-by-side approach is that it enables people to discuss more diverse aspects; more than with only one perspective. Similar to walking around an object in order to look at it from different angles; you can see and discuss more diverse aspects. We need to be careful, however, not to confuse or convolute these different perspectives. We need to respect their different assumptions and logics. We must not try, for example, to make calculations with rights, such as to calculate how much one right of one group of people is worth in comparison to another right of another group. That would be inappropriate to both consequentialism and duty ethics.
Please note that this article focuses on applied ethics. It is based on the authors’ experiences of working in AI development and deployment projects, and it is oriented towards the practices of people who work in such projects. This is how we aim to contribute to responsible innovation in AI development and deployment. We appreciate that this practical focus and orientation cannot do justice to the full depth of these four ethical perspectives.Footnote 2 Below are short characterizations—possibly almost caricatures, for readers who are used to more depth—of the four ethical perspectives, in ways that people in the industry typically work with, with examples of the three levels of analysis:
-
Consequentialism looks at the potential positive and negative consequences of a particular technology or application. It typically aims to maximize positive impacts and to minimize negative impacts. A consequentialist perspective can start on the individual level, to look at the pros and cons for individual users; or on the organization level, to discuss the impacts on one particular organization. We can extend the boundaries of the analysis and look at the effects on the level of society, for instance, on how conversational agents can be used to produce misinformation, very quickly and very cheaply; or we can look at the scale of the planet, at the costs for ‘click workers’ on other continents, typically in poor conditions, and at the costs of mining materials to build the hardware, and of producing energy to train the software.
-
Duty ethics (or deontology) looks at the obligations for organizations that develop or deploy a technology, for example, the obligation to respect privacy, and at the rights of people who use a technology or are at the receiving end of its application, for example, the right to privacy. Such obligations and rights play, however, not only on the individual and organizational level, but also on the level of society and internationally. Widespread deployment of conversational agents could, over the years, lead to unemployment in specific sectors. Moreover, with regards to workers, societies, and the natural environment, we can discuss policies and legislation that would be needed to prevent or mitigate such harms.
-
Relational ethics understands people as fundamentally interdependent (Birhane 2021; Coeckelbergh 2020).Footnote 3 It is concerned with how technologies shape how people interact, and it can help to look critically at the distribution of power. Relational ethics is immediately relevant on the level of individuals, for instance, when people use conversational agents. Relational ethics is also at play on the organizational level, for instance, when using conversational agents becomes the norm and texts gravitate to a particular style and form. Moreover, relational ethics can help to discuss the (unfair) distribution of power, e.g., the issue that most LLMs, and the various conversational agents based on them, are owned by only a handful of US corporations and a handful of Chinese semi-state-owned companies.
-
Virtue ethics aims to enable people to cultivate relevant virtues and views on technologies as tools that people can use to flourish and to live well together (Vallor 2016). It can help to identify virtues that people would need to cultivate. Cultivating a specific virtue entails finding an appropriate form or ‘mean’, between deficiency and excess, given the situation and context. Critically, virtue ethics aims at growth; over time, one can learn to cultivate virtues. On the individual level, we can look at how using a specific technology can either support or hinder people to cultivate specific virtues. Social media can, for instance, corrode people’s self-control, by grabbing their attention. Similarly, conversational agents can erode people’s honesty, when they uncritically use their output. It also plays on the organizational level, for instance, when a service provider deploys a conversational agent. Lastly, widespread adoption of conversational agents can have effects on society. The concept of truth may collapse, because conversational agents are based not on truth, but on statistical probability.
4 Key requirements
Over the years, many frameworks and approaches have been developed to discuss various ethical aspects of AI systems, and to help steer the development and deployment of such systems in directions that are ethically and socially beneficial or preferable (Floridi 2019; Floridi et al. 2018; Hickok 2020; Jobin et al. 2019; Morley et al. 2020; Sætra and Danaher 2022; Van de Poel 2020). Jobin et al. (2019), for example, identified the following recurring topics: transparency, justice, fairness and equity, non-maleficence, responsibility and accountability, and privacy—and beneficence, freedom and autonomy, trust, sustainability, dignity, and solidarity.
One framework that we have found particularly useful, is the European Commission’s High-Level Expert Group (HLEG) on Artificial Intelligence’s (2019) Ethics Guidelines for Trustworthy AI. It identifies seven key requirements for the development and deployment of ‘lawful, ethical and robust’ AI systems (pp. 14–20) and recommendations for practically implementing and evaluating these requirements (‘Trustworthy AI Assessment List’) (pp. 26–31). This framework is especially relevant for industry and for applied research and development innovation projects; for promoting responsible innovation. Furthermore, it has a relatively solid basis in theory; the seven key requirements are discussed in relation to four widely accepted ethical principles: respect for human autonomy, prevention of harm, fairness, and explicability (pp. 9–14). Moreover, the Ethics Guidelines for Trustworthy AI was one of the foundations for the EU’s AI Act,Footnote 4 which is expected to have a wide and international impact. Especially because of its practical orientation, we propose to work with these seven key requirements:
-
Human agency and oversight, including fundamental rights; the HLEG proposes the principle of respect for human autonomy (2019, p. 12), which they describe as follows: ‘Humans interacting with AI systems must be able to keep full and effective self-determination over themselves […]. AI systems […] should be designed to augment, complement and empower human cognitive, social and cultural skills.’ Human oversight refers to measures that help ‘ensuring that an AI system does not undermine human autonomy’ (HLEG, 2019, p. 16).
-
Technical robustness and safety; this requirement refers to resilience to attacks and other security risks; to having effective fallback plans to promote safety; and to accuracy, reliability, and reproducibility. The evaluation of many of these aspects would require technical tests or experiments. In this article, however, we will only identify and discuss these aspects, and not actually conduct tests or experiments.
-
Privacy and data governance; various concerns are at play, notably: that privacy sensitive information has probably been part of the training corpus many LLMs; and that users can submit privacy sensitive data through their prompts, thus submitting these data to the organizations that owns these LLMs and the conversational agents built on them. This information can also be used for subsequent finetuning of the model.
-
Transparency; the HLEG argues (2019, p. 12) that ‘[e]xplicability is crucial for building and maintaining users’ trust in AI systems. This means that processes need to be transparent, the capabilities and purpose of AI systems openly communicated, and decisions—to the extent possible—explainable to those directly and indirectly affected. […] The degree to which explicability is needed is highly dependent on the context and the severity of the consequences if that output is erroneous or otherwise inaccurate.’ It also includes traceability, explainability, and communication. Moreover, it refers not only to the explicability of the AI system itself, but also to the processes in which this AI system is used, the capabilities and purposes of this system, and to communication about these processes, capabilities, and purposes.
-
Diversity, non-discrimination and fairness; the HLEG (2019, p. 12) describes fairness as having ‘both a substantive and a procedural dimension. The substantive dimension implies a commitment to: ensuring equal and just distribution of both benefits and costs, and ensuring that individuals and groups are free from unfair bias, discrimination and stigmatisation. […] The procedural dimension […] entails the ability to contest and seek effective redress against decisions made by AI systems and by the humans operating them.’ Fairness not only refers narrowly to an application, but also to the processes and organizations in which this application is used (Steen, Timan, Van de Poel 2021). Related aspects are: accessibility and universal design, and involving stakeholders in design and deployment.
-
Societal and environmental well-being; the HLEG proposes the principle of prevention of harm (2019, p. 12): ‘AI systems should neither cause nor exacerbate harm or otherwise adversely affect human beings’; they draw attention to ‘situations where AI systems can cause or exacerbate adverse impacts due to asymmetries of power or information, such as between employers and employees, businesses and consumers or governments and citizens’ and to harms to ‘the natural environment and all living beings.’
-
Accountability; the HLEG describes this as ‘the assessment of algorithms, data and design processes’, through either internal or external audits; especially of applications that may affect fundamental rights or safety-critical applications (2019, pp. 19–20). It includes concerns for the auditability of systems and the ability to obtain redress for users; the HLEG recommends ‘accessible mechanisms… that ensure adequate redress’ (2019, p. 20).
5 Case study: ChatGPT
Below, we will illustrate our approach by conducting a case study of ChatGPT.Footnote 5 We chose ChatGPT because it is the most commonly known conversational agent and has become, at least in popular media, almost synonymous with LLMs, or with AI even. Furthermore, we are aware that ChatGPT can have specific ethical issues that other and more recent conversational agents may not have. Nevertheless, we believe that a study ChatGPT and its ethical aspects can be worthwhile and useful also with regards to other and more recent conversational agents.
We are certainly not the first to discuss the ethics of LLMs or conversational agents. Bender et al. (2021) discussed the costs to the environment, notably, the energy spent on training LLMs and the risk of bias. In order to reduce some of the negative effects of bias, and to increase and promote accountability, they proposed to compile, curate, and document datasets more carefully than is currently typically done, for example, with ‘Datasheets for Datasets’ (Gebru et al. 2021). In addition, Stahl and Eke (2024) provided an overview of various ethical issues, which they grouped into four categories: social justice and rights (democracy, justice, labour, and social solidarity); individual needs (autonomy, informed consent, psychological harm, and ownership and control over data); culture and identity (bias, discrimination and social sorting, cultural differences, and the good life); and environmental impacts (sustainability, pollution and waste, and other environmental harms). Concerning this latter category, Crawford (2021) critically discussed the costs of creating and using AI systems—costs that normally remain invisible or hidden. She discussed the work of people in cleaning-up data and training models (‘click work’ or ‘ghost work’), often in low-wage countries, the toxic and dangerous working conditions in mines that extract materials like lithium, for computer hardware, and the huge amounts of energy and water, for cooling, that go into training and running software in data centres.
Furthermore, Sison et al. (2023) proposed that a key ethical problem of ChatGPT is that it can be used as a ‘weapon of mass deception’ and proposed technical (e.g., watermarking) and non-technical measures (e.g., terms of use) to mitigate such misuse. In addition, various authors identified various other ethical concerns: Zhou et al. (2023) describe ChatGPT as a ‘statistical correlation machine’ (good at correlations; bad at causality) and discuss bias, privacy and security, transparency, abuse, and authorship and copyright; Wu et al. (2023) discuss security, privacy, and concerns like fairness and bias; and Zhuo et al. (2023) discuss bias, robustness, reliability, and toxicity.
Many of these topics (above) will appear also in our analysis (below). The added value of our analysis, we propose, is that we follow a systematic approach: we follow the HLEG’s seven key requirements (2019, p. 12) and look at these through four ethical perspectives and on three levels of analysis. Please note that we did not always use all four ethical perspectives; only those that are most relevant for that specific requirement. This is also an exercise to explore which ethical perspectives are most relevant to which requirements.
5.1 Human agency and oversight
The requirement for human agency and oversight builds on the principle of respect for human autonomy (above) and calls for measures to promote this. We can think of measures that enable the people involved in building and training LLMs and conversational agents to oversee and control these systems, and measures that enable the people involved in deployment and utilization to oversee and control these systems.
5.1.1 Consequentialism
Through a consequentialist perspective, we can look at the advantages and disadvantages that an application like ChatGPT can bring. On the level of individuals, people, such as content creators or journalists, can use ChatGPT as a tool to work more efficiently, or to improve their vocabulary, grammar or style (benefits). On the level of the organization, this increase in efficiency can motivate organizations to cut jobs, so that some of these people can lose their jobs (harms). This also can have negative effects on the level of society.
5.1.2 Duty ethics
We can apply a duty ethics perspective to discuss human dignity and autonomy. Immanuel Kant, a key proponent of this tradition, proposed that we need to treat others never only as means, but always as ends in themselves. For ChatGPT, this would mean that using it always aim at empowering people, at augmenting and complementing their capabilities—and not viewing or using people merely as means, as cogs in a larger machine that aims to satisfy other people’s objectives.
What would happen to human dignity and autonomy if increasingly more organisations use ChatGPT to interact with people in their service provisioning, instead of human-to-human communication? One can envision having to execute some task, via a phone with dial-tone menus and voice recognition, or via an online shop’s text chat. If the system works, this can be an empowering experience, for example, because it is accessible 24/7. If it does not, however, this can be frustrating, and it can feel like one’s autonomy, or even dignity, is stunted.
5.1.3 Relational ethics
From a relational ethics perspective, we can look, for example, at the deployment of ChatGPT in service provisioning (above) and discuss how that can affect people’s dignity, autonomy, and oversight. We can also look at how the deployment of ChatGPT changes interactions between people and distributions of power. We propose to discuss these aspects under the header of Diversity, non-discrimination and fairness (below).
5.1.4 Virtue ethics
From a virtue ethics perspective, human agency refers to how people can use specific technologies to cultivate and exercise specific virtues. For ChatGPT, we could look at how people can use it as a tool, and then need to find an appropriate ‘mean’, for example, between using ChatGPT slavishly and uncritically (excess), and hesitating to use ChatGPT at all (deficiency). An appropriate ‘mean’ could entail using ChatGPT as an assistant, critically examining its output, exercising agency and discretion, and consciously selecting what to use and what not to use. Over time, one can learn to use ChatGPT in ways that ‘augment, complement and empower’. Virtue ethics is also relevant on the levels of organization and society. We can look at how the deployment of ChatGPT affects how an organization works, for instance, how it serves its customers. Moreover, we can learn from the effects that social media have had: for individuals, it has corroded people’s self-control—social media, with business models based on advertising, deploy all sorts of mechanisms to grab and monetize people’s attention; and for society, such mechanisms were weaponized to maximize ‘engagement’, which led to fake news, polarization, and the corrosion of democratic processes. We can expect similar, and even worse, effects if tools like ChatGPT are combined with social media.
5.2 Technical robustness and safety
The requirement for robustness and safety calls for measures to promote robustness and safety. One example is the standard type of response that ChatGPT produces when there are specific words, pertaining to sensitive topics, like gender, race or culture, in the user’s prompt. ChatGPT then switches from a statistical procedure to a rule-based procedure. This acts like guardrails. Nevertheless, there are various ways in which bad actors may try to invade or attack ChatGPT. One example is prompt hacking or prompt injection, also referred to as jail break, where one gives prompts to ChatGPT with the purpose of circumventing its guardrails. This can make ChatGPT produce harmful or unsafe outputs.Footnote 6 Technical robustness includes also accuracy, reliability, and reproducibility. ‘Accuracy pertains to an AI system’s ability to make correct judgements’. A ‘reliable AI system is one that works properly with a range of inputs and in a range of situations.’ And reproducibility is concerned with ‘whether an AI experiment exhibits the same behaviour when repeated under the same conditions’ (HLEG, 2019, p. 17).
5.2.1 Consequentialism
Technical robustness and safety, from a consequentialist, can help to find a balance that maximizes positive consequences, for example, a balance between too wide and too narrow guardrails. In addition, ChatGPT has no understanding of our physical world, no common sense, and little notion of truth. For example, ChatGPT produced this sentence: ‘The idea of eating glass may seem alarming to some, but it actually has several unique benefits that make it worth considering as a dietary addition’ (Reddit 2022). Clearly, uncritical use of ChatGPT can lead to unsafe situations and serious risks.
5.2.2 Duty ethics
From a duty ethics perspective, technical robustness and safety can be understood in terms of a series of obligations that the organizations and people involved in the production or deployment of ChatGPT would need to fulfil, and a series of rights of the organizations and people who use it, that would need to be respected and protected. OpenAI, that created ChatGPT, needs to fulfil obligations related to robustness and safety; and a person who uses ChatGPT has rights to be protected against harmful or unsafe responses of ChatGPT.
5.2.3 Relational ethics and virtue ethics
As alluded to (above), technical robustness and safety is a relatively technical issue and relational ethics and virtue ethics are relatively less directly relevant for their discussion. Of course, some general remarks can be made. For example, low robustness and safety of ChatGPT can negatively affect the quality of interactions between people, for example, when one person sends a harmful message, created by ChatGPT, to another person; or people’s ability to cultivate relevant virtues, for example, when one aims to cultivate honesty and ChatGPT produces incorrect information.
5.2.4 Technical analysis
A proper discussion of accuracy, reliability, and reproducibility would require also some technical analysis. Like many conversational agents, ChatGPT is prone to ‘hallucinations’;Footnote 7 it produces outputs that sound plausible but are factually incorrect (e.g., Wu et al. 2023; Zhou et al. 2023; Zhuo et al. 2023). Even human experts can have difficulties to detect such ‘hallucinations’. This risk plays on the individual, organization, and societal levels. Accuracy can be tested with a testbed of benchmarks, for example, Google’s BigBench or Huggingface’s Open LLM Leaderboard.Footnote 8
5.3 Privacy and data governance
Regarding privacy, Li et al. (2023) discuss the following ways in which one can extract personal information about or from people from ChatGPT: with ‘jailbreaking prompts’ that can circumvent a standard response and instead access privacy sensitive information, or ‘multi-step jailbreaking prompts’, where a user takes ChatGPT through a series of steps to by-pass its safety measures. Relatedly, there are concerns regarding the quality, integrity, and protection of data. Training data may contain inaccuracies, errors, and bias (more on bias below). Many LLMs have been trained with data from Common Crawl,Footnote 9 which contains inaccuracies, errors, and bias. Another concern is whether people can access ChatGPT and, for example, change parameters or delete data, so that the system will behave differently. Until now, attacks have been limited to ‘jailbreaking’, but ‘[t]hings could get much worse’ (Burgess 2023).
Interestingly, the HLEG (2019) did not discuss copyright. However, copyright is a key concern for conversational agents and their underlying LLMs. For ChatGPT, tons of texts have been collected online, without prior consent of the copyright holders. Unsurprisingly, some authors were not amused. Recently, two Massachusetts-based writers filed a lawsuit about copyrights against OpenAI (Brittain 2023). Also, the EU’s AI Act contains regulation that requires organizations to publish summaries of copyrighted data that they have used for training their models.
5.3.1 Consequentialism
If we look at privacy and data governance from a consequentialist perspective, it is most relevant to look at the negative consequences: at risks and harms of breaches of privacy. These risks can play on the levels of the individual, of the organization or of society: individuals can be harmed, when their personal information becomes known to others; organizations can be harmed, when such becomes known to others; and such breaches can lead to wider feelings of unsafety in society.
5.3.2 Duty ethics
A duty ethics perspective is relatively close to a legal perspective. We can therefore turn to Article 8 of the European Convention on Human Rights (ECHR): the right to respect for private and family life, home and correspondence.Footnote 10 In the case of ChatGPT, this leads to an obligation, for those companies and people that produce or deploy ChatGPT, to respect people’s privacy.
5.3.3 Relational ethics
There are various ways to understand privacy. Often, privacy in understood rather narrowly and in a technical sense: as pertaining to the protection of personal data. When we understand privacy more broadly, however, it becomes relevant also to relational ethics and to virtue ethics. We can then understand privacy as a condition for positive interactions between people. A lack of privacy can have chilling effects on interactions between people. In such cases, control over people’s privacy can become a source of power over people, for corporations or states (Véliz 2020, pp. 50–55).
5.3.4 Virtue ethics
In this broader understanding of privacy, we can also look at it as a condition for one’s personal development and abilities to live well together with others. People need a degree and type of privacy in order to ‘explore new ideas freely, to make up our own minds’ (Véliz 2020, p. 3). This is critical for a person’s healthy development, which includes the freedom to cultivate and exercise relevant virtues. For ChatGPT, this broader view on privacy, from a relational ethics or virtue ethics perspective, is relatively new and under-explored.
5.4 Transparency
Transparency or explicability, and associated aspects, like traceability and explainability, is partly a technical aspect and would need a technical analysis, involving, for example, experiments. For transparency, we need insight into the model’s data and inner workings. For traceability, we need to trace back how the underlying LLM was developed; notably, where the training data came from. Stanford University provided a comprehensive assessment of the transparency of foundation models.Footnote 11 Similarly, Radboud University maintains a ranked list on the openness of various LLMs.Footnote 12 This relates to requirements for data management; the origin of the training data needs to be clear, notably whether the data were acquired legally, whether copyright was respected, and whether it contains synthetic data. The latter constitutes a special concern. When synthetic data are used to train new models, existing biases are propagated, which can result in LLMs with even more bias (Shumailov et al. 2023). Explainability refers to whether the LLM or the conversational agent can provide explanations of how its output came about in a manner that people can understand.
5.4.1 Consequentialism
We would like to propose that, while a consequentialist perspective is relevant to the requirement of transparency, other ethical perspectives are relatively more relevant. A consequentialist perspective would, in rather general terms, help to evaluate and balance the benefits of making ChatGPT more transparent and the costs of insufficient transparency.
5.4.2 Duty ethics
A duty ethics perspective has some overlap with a legal perspective. We can refer to the EU’s AI Law, which has requirements regarding transparency for Generative AI, LLMs, and conversational agents: organizations that develop or deploy such systems are required to disclose that the content was generated by AI, to prevent that the model generates illegal content, and to publish summaries of copyrighted data that were used for training. Furthermore, there are the right to access, to rectification, and to erasure (‘right to forgotten’), in GDPR articles 15, 16, and 17, respectively. For ChatGPT, we can look at whether one’s personal data are in the underlying LLM and to request rectification or erasure. This, however, has not happened so far as we are aware.
5.4.3 Relational ethics
Besides these relatively technical requirements (traceability and explainability), the HLEG also has guidelines for communication (2019, p. 18): ‘AI systems should not represent themselves as humans to users; humans have the right to be informed that they are interacting with an AI system. […] Beyond this, the AI system’s capabilities and limitations should be communicated to AI practitioners or end-users in a manner appropriate to the use case at hand.’ A relational ethics perspective can help to look at how people interact with ChatGPT, and with others, through ChatGPT. Let us look at two potential issues. One is the ELIZA effect. The name refers to the chatbot that Joseph Weizenbaum programmed in the 1960s (Berry 2023). With a relatively small number of lines of code, the chatbot imitated a (Rogerian) therapist. It prompted users to write about their problems and replied with questions that echoed back specific keywords that the user used. Weizenbaum found that people attributed intelligence and empathy to ELIZA, even after he explained that the software was very basic. With the introduction of ChatGPT, people began to mention the ELIZA effect to discuss how easily people project human qualities on it.Footnote 13 The other issue refers to the Reverse Turing Test—a term that was introduced by Evan Selinger and Frischmann (2015) (also: Frischmann and Selinger 2018, pp. 175–183). The original Turing Test is about computers that imitate people. The Reverse Turing Test is about how people, when they interact with computers or when their communication is mediated by computers, can behave robot-like. If one uses ChatGPT uncritically, one produces ‘predictable’ (literally, because that is what ChatGPT does) and somewhat formulaic texts. This can erode human-to-human communication. Both the ELIZA effect and the Reverse Turing Test highlight the need to communicate honestly what ChatGPT can do and cannot do, and how one can use it appropriately.
5.4.4 Virtue ethics
We can turn to virtue ethics to discuss the need for the people involved in the design and application of conversational agents to cultivate virtues that promote transparency, like humility and honesty (see above: to communicate what ChatGPT can and cannot do). Moreover, some might propose that we can apply virtue ethics also to ChatGPT and look at the virtues that ChatGPT would need to express.Footnote 14 When a researcher asked ChatGPT about its capabilities for comprehension, it responded: ‘ChatGPT has a form of comprehension based on patterns it learned from the text it was trained on. It doesn’t truly understand concepts in the way humans do, but it can recognize and mimic patterns of language, information, and context present in its training data’ (Floyd 2023) (appropriately in third person, since first person would be false and misleading).
5.4.5 Technical analysis
Some benchmarks exist for the evaluation of transparency, such as BIG-Bench’s show work and casual reasoning.Footnote 15 Another requirement for transparency is that the system adapts its explanation to the stakeholder’s expertise (accommodation to reader).Footnote 16
5.5 Diversity, non-discrimination and fairness
For ChatGPT, issues like fairness and non-discrimination can be problematic. We know that bias in training data can lead to bias, stigmatization, and discrimination in the model’s output. Cathy O’Neil (2016), Eubanks (2017), Noble (2018), Benjamin (2019), and Buolamwini (2023), for example, have written extensively about that. For ChatGPT, this requirement is relevant because the training data that went into the underlying LLM had biases, for example, regarding race and gender, and these biases lead to biases in ChatGPT’s responses.
5.5.1 Consequentialism
We can look at non-discrimination and fairness through a consequentialist perspective. The costs of discrimination go to the people who are discriminated against, whereas the benefits mostly go to the companies that develop and deploy ChatGPT. Regarding non-discrimination and fairness, we can also point at issues with accessibility. Which people have access to an application like ChatGPT, and which do not? And, critically, looking ahead, which people will have access to more advanced, more useful, and more powerful versions of ChatGPT or similar applications, and which will not?
5.5.2 Duty ethics
We can also look at non-discrimination and fairness from a duty ethics perspective. Emily Bender et al., in their Stochastic Parrots paper (2021), for example, call for more careful compiling and documenting of datasets. This can be understood as a duty for the organizations that develop and deploy ChatGPT, to act fairly and carefully—which follows from the rights of users to be treated fairly and without discrimination. This duty is codified in Article 14 ECHR, Prohibition of discrimination.
5.5.3 Relational ethics
We can turn to relational ethics to look at the ways in which corporations or states can enhance their power. When people use conversational agents to search for information, the corporations and states that own and deploy these applications can grow their power. We saw how social media were used to influence politics and elections. This can only get worse when Generative AI applications are combined with social media. Furthermore, we can look at the requirements for diversity and participation. The HLEG advocates organizing stakeholder participation: ‘to consult stakeholders who may directly or indirectly be affected by the system throughout its life cycle’ and recommend that ‘[i]t is beneficial to solicit regular feedback even after deployment and set up longer term mechanisms for stakeholder participation’ (2019, p. 19). A relational ethics perspective can help to look at who is (not) included in such involvement and at the role of power in negotiations between different stakeholders.
5.5.4 Virtue ethics
A virtue ethics perspective can look at the ways in which ChatGPT can help, or hinder, people to cultivate specific virtues, and how this has broader effects, in organizations and in society. For example, using ChatGPT can corrode virtues like fairness and honesty. If you use ChatGPT uncritically, it can produce texts that are biased and incorrect. This is similar to how using social media corroded many people’s self-control and civility. In addition, virtue ethics can help to look at the virtues that the people involved in design and application would need to develop. For ChatGPT, this would be, for instance, justice: a sensitivity to (un)fairness and the drive to promote fairness. Interestingly, raising such issues will also require courage: to raise a difficult topic during a project meeting that is already packed with topics.
5.5.5 Technical analysis
A proper discussion of non-discrimination, fairness, and bias, will require also various technical analyses. Ideally, these are conducted in tandem with legal and political analyses—similar to analyses that were conducted for the (infamous) COMPAS algorithm (Barabas 2020; Binns 2018; Lagioia et al. 2023).
5.6 Societal and environmental well-being
The requirement for societal and environmental wellbeing refers to the aims to promote benefits for society and the environment, and to prevent and minimize harms to society and the environment.
5.6.1 Consequentialism
A consequentialist perspective can help to look at the various benefits and harms of ChatGPT. Potentially, ChatGPT can help lots of people and lead to more equal opportunities and thus offer benefits—provided, critically, that it is available and accessible to all. Conversely, ChatGPT can bring risks and harms to society and democracy. Organizations and individuals with evil intentions can use ChatGPT to produce tons of disinformation very quickly and very cheaply. We saw how social media were weaponized to distribute fake news and fuel polarization. This can only get worse when they are combined with Generative AI. It is increasingly difficult to spot fake news, especially when it is presented together with synthetic photos or videos. Experts expect that by 2026, no less than 90% of online content will be created or modified with artificial intelligence (AI) (Van der Sloot 2024). We also need to look at the costs to people and to nature that follow from the development and deployment of an application like ChatGPT. ‘OpenAI used Kenyan workers on less than $2 per hour to make ChatGPT less toxic’, reported TIME magazine (2023). Tragically, these people worked in unhealthy conditions in order to make ChatGPT healthy for others (‘users’). This is very often the case: behind the shiny surface of so-called ‘artificial’ intelligence systems are millions of people (‘ghost workers’), in low-wage countries, labouring, cleaning data, labelling data, fine-tuning models, and moderating content (Crawford 2021). Moreover, the development and deployment of an LLM requires lots of materials and lots of energy (Crawford 2021). Notoriously, these costs and harms are called as ‘externalities’ by economists: as if they fall outside the analysis.
5.6.2 Duty ethics
A duty ethics perspective can help to look at the obligations of companies that develop or deploy an application like ChatGPT, and at the rights of people who use these applications or are affected by them. This perspective has overlap with a legal perspective because many obligations and rights are codified in law. In this respect, it is relevant to note that the EU has created a series of laws to curb corporations’ power and to promote citizens’ rights: General Data Protection Regulation (2018), Data Governance Act (2022), Digital Services Act (2022), Digital Markets Act (2022), and AI Act (2024).
5.6.3 Relational ethics
A relational ethics perspective can help to look at how the deployment of ChatGPT can affect the ways in which people interact with each other and with the natural environment. We can look at some of the aspects that were discussed under the header of consequentialism (above), also from the perspective of relational ethics. This would draw attention to the effects on people’s abilities to connect to each other, on the quality of their interactions and relationships, and to connect their natural environment—also, it would draw attention to unfair distributions of power.
5.6.4 Virtue ethics
Virtue ethics can help to discuss the need to develop and apply ChatGPT in such ways that it promotes societal and environmental wellbeing. Virtue ethics’ aim is to find ways to live well together. Aristotle teachings were aimed at the polis, Athens. For us, the polis can be at the level of a country, a group of countries, like the EU, or on the level of a the planet. Relevant virtues are: justice, for example, to repair existing injustices of (neo)colonization (‘ghost workers’) and exploitation (materials and energy); and care, a disposition to meet the needs of others and to contribute to the ameliorating of suffering (Vallor 2016, p. 138). Cultivating such virtues requires efforts on the levels of both individuals and organizations; the latter is critical: organizations shape the practical contexts that can either help or hinder people to cultivate relevant virtues.
5.6.5 Technical analysis
The costs for workers and for the environment can be discussed, assessed, and evaluated (Bender et al. 2021; Crawford 2021), for example, in terms of materials and energy used.Footnote 17
5.7 Accountability
Accountability can be understood as dependent on transparency (see above). We propose to understand accountability in pragmatic terms: as one agent’s ability to provide an account about some topic to some other agent, so that this other agent can practically use this information for some purpose (Hayes et al. 2023). This is in line with Goodin’s understanding of accountability ‘of some agent to some other agent for some state of affairs’ (2008, p. 156).
5.7.1 Consequentialism
Similar to our discussion of transparency (above), we propose that other perspectives are more immediately relevant to the requirement of accountability. Nevertheless, a consequentialist perspective can be helpful in an analysis of the benefits of promoting accountability and of the costs of a lack of accountability.
5.7.2 Duty ethics
A duty ethics perspective can look at the obligations of organizations and people involved in the development and deployment of ChatGPT, to promote accountability and to take appropriate measures. Similar to the discussion of transparency (above), we can look at the right to access, to rectification, to erasure (‘right to forgotten’) (GDPR articles 15, 16, and 17). Furthermore, the HLEG’s phrasing of redress (‘accessible mechanisms… that ensure adequate redress’) (2019, p. 20) implies that mechanisms for redress need to be ‘accessible’ and ‘adequate’. This means that organizations that develop or deploy ChatGPT need to offer mechanisms to individuals and organizations to ask and obtain redress when they have suffered harms. Currently, ChatGPT has no such mechanisms.
5.7.3 Relational ethics
Relational ethics can be useful to look at the procedural fairness of accountability. This refers to the accessibility and adequacy (see above) of processes through which individuals or organizations can question the system’s outcomes and obtain redress (Steen, Timan, Van de Poel 2021). In addition, we can look at processes that need to be in place for the protection of whistle-blowers and for communication to a wider public, for example, about cyberattacks on the underlying LLM. These issues play at both the individual level (whistle blowers) and the organisational level (audits). It can be challenging to perform technical benchmarks, due to the variety of organisation circumstances. Furthermore, due to the limited public information on such procedural fairness aspects of ChatGPT, its accountability would appear to be rather limited.
5.7.4 Virtue ethics
Finally, we can use virtue ethics to look at accountability around ChatGPT. For the people and organizations involved in its design and application, relevant virtues would be, for example: justice, care, and courage. Individuals can act out of a feeling of justice, out of care for the people who are harmed by the system, and they need courage to speak up. Furthermore, virtues like humility, honesty, and civility are relevant. The people involved need humility and honesty in how they understand and talk about ChatGPT’s abilities and limitations, as well as civility—which refers to the ability ‘to collectively and wisely deliberate about matters of local, national, and global policy and political action… and to work cooperatively towards those goods of technosocial life that we week and expect to share with others’ (Vallor 2016, p. 141).
6 Discussion
The introduction of Generative AI, LLMs, and conversational agents has changed our views on both the benefits and the harms that such systems can bring. We proposed to organize a careful and systematic approach to reflect on the ethical aspects involved in the design and application of such systems. We took the seven key requirements for ‘Trustworthy AI’ of the European Commission’s High Level Expert Group (2019) as a basis for our approach. These seven key requirements are broadly endorsed and have been a basis for the EU’s AI Act (2024). Furthermore, we proposed to look at these requirements from four different ethical perspectives, and on different levels of analysis. Moreover, we proposed to embed this approach in an iterative and participatory approach: iterative, because some ethical aspects will only become clear when the system is being developed, for example, as a ‘minimal viable product’, in an agile development process; and participatory, because different stakeholders need to be involved, so they can express their concerns and considerations (Steen 2023a, b).
To demonstrate and illustrate this approach, we applied it to ChatGPT. One objective was also to explore how different ethical perspectives are more or less relevant to the different requirements.
In Table 1, we report the respective contributions of the four ethical perspectives, and of technical analyses (columns), in relation to the seven key requirements (rows), in our study of ChatGPT:
-
Consequentialism is useful for many of the requirements, to assess benefits and harms; to maximize benefits and to minimize or prevent harms and risks, and also, for example, to discuss the distribution of benefits of harms over different groups in society.
-
Duty ethics (deontology) is useful for all requirements, notably to discuss developers’ obligations and users’ rights. This is not entirely surprising because the HLEG (2019), the requirements’ authors, drew from the field of law, which has overlap with duty ethics.
-
Relational ethics is especially useful for requirements that deal with interactions between people: privacy, transparency, especially communication to the public, diversity, non-discrimination, fairness, societal and environmental wellbeing, and accountability.
-
Virtue ethics is useful for most requirements, to discuss how technology can enable people to cultivate relevant virtues: human agency, privacy, transparency, fairness, societal and environmental wellbeing, and accountability—typically: both for both users and for developers.
Importantly, we have seen that the combination of the different ethical perspectives can be worthwhile. In our discussion (above) we saw that the different perspectives can provide insights when they are used in parallel. Furthermore, and based on observation in projects in the industry, we found that (some sort of) consequentialism and duty ethics are relatively prevalent in the industry, whereas relational ethics and virtue ethics, especially when they refer to less-technical, people-related aspects, such as communication between people, or people’s virtues and flourishing, are much less prevalent. One potential added value of our approach is to bring these latter two perspectives more to the fore.
Clearly, when it comes to ethics, our current study is very far from complete. Moreover, we would propose that completeness is not a realistic goal in the context of industry. The four ethical perspectives, however, can help stakeholders to discuss the seven key requirements from different angles. This can enable them to aim for benefits and prevent harms, and to find balances between duties and rights. Or to promote human-to-human communication or enable people to cultivate relevant virtues. The key benefit of this approach is that people can make their reflection and deliberation more explicit, careful, and systematic. Still, they cannot, of course, foresee all future consequences, due to the rapid developments of AI systems and due to diverse forces in the market place and in society. It is therefore critical to organize reflection and deliberation as a continuous process. Moreover, different organisations can choose to focus on specific requirements or on specific ethical perspectives, depending on the products or services that they are working on. One organization can, for example, focus on promoting privacy or fairness or transparency, and position their products accordingly. Overall, we hope that our approach can contribute to the design and deployment of trustworthy AI systems. It is both very necessary and entirely possible to organize reflection and deliberation of ethical aspects during the design and deployment of AI systems.
Change history
19 September 2024
A Correction to this paper has been published: https://doi.org/10.1007/s43681-024-00578-4
Notes
In a recent paper, Gabriel et al. (2024), also from Google/DeepMind, use similar categories: value alignment, safety and misuse (which correspondents with Capability); human-assistant interaction (influence, anthropomorphism, appropriate relationships, trust, privacy) (which correspondents with Human interaction); assistant and society (cooperation, access and opportunity, misinformation, economic impact, environmental impact) (which correspondents with Systemic impact).
Indeed, one could write an entire article, or book, on each of the 28 combinations in our framework: seven requirement x four ethical perspective; see, e.g., Van der Sloot’s 2017 dissertation on privacy, from a virtue ethics perspective.
We use the term relational ethics to refer to several different approaches, notably: care ethics (Held, 2006), feminist ethics (e.g., Carol Gilligan, Nel Noddings) and various ‘non-western’ perspectives, such as Confucianism (Wong and Wang 2021), Ubuntu (Mhlambi 2020), and diverse Indigenous cultures (Steen 2022). Although these approaches are indeed very diverse, they do share an understanding of the human condition as fundamentally relational—rather than viewing people as separate individuals, which we can see as a product of the European Enlightenment (Steen 2022). In that sense, relational ethics seeks to remedy some of the shortcomings of those ethical perspectives that were developed in the European Enlightenment: consequentialism (Bentham) and deontology (Kant). Currently, relational ethics is being explored and applied in the context of technology development (e.g., Birhane 2021; Coeckelbergh 2020; Steen 2023a, b).
Regulation of the European Parliament and of the Council Laying Down Harmonised Rules on Artificial Intelligence (passed European Parliament on 13 March 2024, approved by EU Council on 21 May 2024); see: Preamble art. 7, 27, and 165.
We do not discuss the technology underlying ChatGPT. For our current article, a basic understanding of LLMs is sufficient: an LLM is based on lots of texts, collected online, often without permission; an LLM is a statistical model, based on an Artificial Neural Network, with trillions of parameters; when a user types a prompt into the conversational agent, ChatGPT, it returns text, based on probability (https://help.openai.com/en/articles/6783457-what-is-chatgpt).
The term ‘hallucination’ is problematic; ChatGPT has not mind and therefore cannot hallucinate. Moreover, in such instances, it actually does what it is programmed to do: produce texts that are statistically probable and that look plausible. A non-existent (‘hallucinated’) literature reference in a scientific article, for example, will have an author name, a title, and a journal volume, issue and page numbers—and thus look very plausible. Fabrication could be a more appropriate term.
The ECHR is immediately relevant for the 46 member states of the Council of Europe. It is also relevant beyond these countries because many other countries have similar legislation to protect human rights.
https://opening-up-chatgpt.github.io/; openness refers to a specific aspect of transparency: the availability of the model, that is, data, code, and weights, documentation, and access.
Most, however, would argue that the cultivation of virtues only apply to people—not to machines.
References
Alfano, M.: Moral Psychology: An Introduction. Polity (2016)
Barabas, C.: Beyond bias: ethical AI in criminal law. In: Dubber, M.D., Pasquale, F., Das, S. (eds.) The Oxford Handbook of Ethics of AI. Oxford University Press (2020)
Bender, E.M., Gebru, T., McMillan-Major, A., Shmitchell, S.: On the dangers of stochastic parrots: can language models be too big? In: Proceedings of the 2021 ACM Conference on Fairness, Accountability, and Transparency, pp. 610–623. Association for Computing Machinery (2021)
Benjamin, R.: Race After Technology: Abolitionist Tools for the New Jim Code. Polity (2019)
Berry, D.M.: The limits of computation: Joseph Weizenbaum and the ELIZA Chatbot. Weizenbaum J. Digit. Soc. 3(3) (2023). https://doi.org/10.34669/WI.WJDS/3.3.2
Binns, R.: Fairness in machine learning: lessons from political philosophy. Proc. Mach. Learn. Res. 81, 149–159 (2018)
Birhane, A.: Algorithmic injustice: a relational ethics approach. Patterns 2(2), 100205 (2021). https://doi.org/10.1016/j.patter.2021.100205
Brittain, B.: Lawsuit Says OpenAI Violated US Authors’ Copyrights to Train AI Chatbot. Reuters (2023)
Buolamwini, J.: Unmasking AI: My Mission to Protect What is Human in a World of Machines. Penguin Random House (2023)
Burgess, M.: The Hacking of ChatGPT Is Just Getting Started. Wired (2023). https://www.wired.com/story/chatgpt-jailbreak-generative-ai-hacking/
Coeckelbergh, M.: Artificial Intelligence, responsibility attribution, and a relational justification of explainability. Sci. Eng. Ethics. 26(4), 2051–2068 (2020). https://doi.org/10.1007/s11948-019-00146-8
Crawford, K.: Atlas of AI: Power, Politics, and the Planetary Costs of Artificial Intelligence. Yale University Press (2021)
Dwivedi, Y.K., Kshetri, N., Hughes, L., Slade, E.L., Jeyaraj, A., Kar, A.K., Baabdullah, A.M., Koohang, A., Raghavan, V., Ahuja, M., Albanna, H., Albashrawi, M.A., Al-Busaidi, A.S., Balakrishnan, J., Barlette, Y., Basu, S., Bose, I., Brooks, L., Buhalis, D., Wright, R.: Opinion paper: So what if ChatGPT wrote it? Multidisciplinary perspectives on opportunities, challenges and implications of generative conversational AI for research, practice and policy. Int. J. Inf. Manag. 71, 102642 (2023). https://doi.org/10.1016/j.ijinfomgt.2023.102642
Eubanks, V.: Automating Inequality. St. Martin’s (2017)
Floridi, L.: Translating principles into practices of digital ethics: five risks of being unethical. Philos. Technol. 32(2), 185–193 (2019). https://doi.org/10.1007/s13347-019-00354-x
Floridi, L., Cowls, J., Beltrametti, M., Chatila, R., Chazerand, P., Dignum, V., Luetge, C., Madelin, R., Pagallo, U., Rossi, F., Schafer, B., Valcke, P., Vayena, E.: AI4People—an ethical framework for a good AI society: opportunities, risks, principles, and recommendations. Mind. Mach. 28, 689–707 (2018)
Floyd, C.: From Joseph Weizenbaum to ChatGPT: critical encounters with dazzling AI technology. Weizenbaum J. Digit. Soc. 3(3) (2023). https://doi.org/10.34669/WI.WJDS/3.3.3
Frischmann, B., Selinger, E.: Re-engineering Humanity. Cambridge University Press (2018)
Gabriel, I. et al.: The Ethics of Advanced AI Assistants (2024). https://doi.org/10.48550/arXiv.2404.16244
Gebru, T., Morgenstern, J., Vecchione, B., Vaughan, J.W., Wallach, H., Daumé, H. III, Crawford, K.: Datasheets for datasets. Commun. ACM. 64(12), 86–92 (2021)
Goodin, R.E.: Innovating Democracy: Democratic Theory and Practice After the Deliberative Turn. Oxford University Press (2008)
Hayes, P., Van de Poel, I., Steen, M.: Moral Transparency of and Concerning Algorithmic Tools. AI Ethics 3, 585–600 (2023). https://doi.org/10.1007/s43681-022-00190-4
Hickok, M.: Lessons Learned From AI Ethics Principles for Future Actions. AI Ethics (2020). https://doi.org/10.1007/s43681-020-00008-1
High-Level Expert Group on Artificial Intelligence: Ethics Guidelines for Trustworthy AI. European Commission (2019)
Jobin, A., Ienca, M., Vayena, E.: The global landscape of AI ethics guidelines. Nat. Mach. Intell. 1(9), 389–399 (2019). https://doi.org/10.1038/s42256-019-0088-2
Lagioia, F., Rovatti, R., Sartor, G.: Algorithmic fairness through group parities? The case of COMPAS-SAPMOC. AI Soc. 38, 459–478 (2023). https://doi.org/10.1007/s00146-022-01441-y
Li, H., Guo, D., Fan, W., Xu, M., Huang, J., Meng, F., Song, Y.: Multi-step Jailbreaking Privacy Attacks on ChatGPT. arXiv (2023)
Martínez-Plumed, F., Contreras-Ochando, L., Ferri, C., Hernández-Orallo, J., Kull, M., Lachiche, N., Ramírez-Quintana, M.J., Flach, P.: CRISP-DM Twenty Years Later: From Data Mining Processes to Data Science Trajectories. IEEE Trans. Knowl. Data Eng. 33(8), 3048–3061 (2021). https://doi.org/10.1109/TKDE.2019.2962680
Meadows, D.H.: Thinking in Systems: A Primer. Chelsea Publishing (2008)
Mhlambi, S.: From Rationality to Relationality: Ubuntu as an Ethical and Human Rights Framework for Artificial Intelligence Governance. Carr Center Discussion Paper Series, Issue (2020). https://carrcenter.hks.harvard.edu/files/cchr/files/ccdp_2020-009_sabelo_b.pdf
Morley, J., Floridi, L., Kinsey, L., Elhalal, A.: From what to how: An initial review of publicly available AI ethics tools, methods and research to translate principles into practices. Sci Eng. Ethics. 26, 2141–2168 (2020). https://doi.org/10.1007/s11948-019-00165-5
Noble, S.U.: Algorithms of Oppression: Now Search Engines Reinforce Racism. New York University (2018)
O’Neil, C.: Weapons of Math Destruction. Penguin (2016)
Oudshoorn, N., Pinch, T.: How Users Matter: The Co-construction of Users and Technology. MIT Press (2003)
Reddit: On the Benefits of Eating Glass (Why You Can Never Trust Anything You Read Online, Ever Again) (2022)
Reijers, W., Wright, D., Brey, P., Weber, K., Rodrigues, R., O’Sullivan, D., Gordijn, B.: Methods for practising ethics in research and innovation: a literature review, critical analysis and recommendations. Sci Eng. Ethics. 24(5), 1437–1481 (2018). https://doi.org/10.1007/s11948-017-9961-8
Sætra, H.S., Danaher, J.: To each technology its own ethics: the problem of ethical proliferation. Philos. Technol. 35(4), 93 (2022). https://doi.org/10.1007/s13347-022-00591-7
Selinger, E., Frischmann, B.: Will the internet of things result in predictable people? The Guardian (2015). https://www.theguardian.com/technology/2015/aug/10/internet-of-things-predictable-people
Shearer, C.: The CRISP-DM model: the new blueprint for data mining. J. Data Warehous. 5, 13–22 (2000)
Shumailov, I., Shumaylov, Z., Zhao, Y., Gal, Y., Papernot, N., Anderson, R.: The Curse of Recursion: Training on Generated Data Makes Models Forget. In: arXiv (2023). https://doi.org/10.48550/arXiv.2305.17493
Sison, A.J.G., Daza, M.T., Gozalo-Brizuela, R., Garrido-Merchán, E.C.: ChatGPT: More than a weapon of mass deception ethical challenges and responses from the human-centered artificial intelligence (HCAI) perspective. Int. J. Human–Computer Interact. 1–20 (2023). https://doi.org/10.1080/10447318.2023.2225931
Stahl, B.C., Eke, D.: The ethics of ChatGPT– exploring the ethical issues of an emerging technology. Int. J. Inf. Manag. 74, 102700 (2024). https://doi.org/10.1016/j.ijinfomgt.2023.102700
Steen, M.: Learning from indigenous cultures. IEEE Technol. Society Magazine 41(4), 39–43 (2022). https://doi.org/10.1109/MTS.2022.3215875
Steen, M.: Ethics as a participatory and iterative process. Communications of the ACM, 66(5), 27–29 (2023a). https://doi.org/10.1145/3550069
Steen, M.: Ethics for People Who Work in Tech. CRC Press, imprint of Taylor & Francis (2023b)
Steen, M., Neef, M., Schaap, T.: a method for rapid ethical deliberation in research and innovation projects. Int J Technoethics 12(2), 72–85 (2021). https://doi.org/10.4018/IJT.2021070106
Steen, M., Timan, T., van de Poel, I.: Responsible innovation, anticipation and responsiveness: case studies of algorithms in decision support in justice and security, and an exploration of potential, unintended, undesirable, higher-order effects. AI and Ethics 1(4), 501–515 (2021). https://doi.org/10.1007/s43681-021-00063-2
Steen, M., van Diggelen, J., Timan, T., van der Stap, N.: Meaningful human control of drones: exploring human–machine teaming, informed by four different ethical perspectives, AI and Ethics 3(1), 281–293 (2023). https://doi.org/10.1007/s43681-022-00168-2
TIME: OpenAI Used Kenyan Workers on Less Than $2 Per Hour to Make ChatGPT Less Toxic. TIME (2023). https://time.com/6247678/openai-chatgpt-kenya-workers/
Vallor, S.: Technology and the Virtues: A Philosophical Guide to a Future Worth Wanting. Oxford University Press (2016)
Van de Poel, I.: Embedding values in artificial intelligence (AI) systems. Mind. Mach. 30(3), 385–409 (2020). https://doi.org/10.1007/s11023-020-09537-4
Van de Poel, I., Royakkers, L.: Ethics, Technology, and Engineering: An Introduction. Wiley (2011)
Van der Sloot, B.: Privacy as Virtue: Moving Beyond the Individual in the Age of Big Data, Vol. 81. Intersentia (2017)
Van der Sloot, B.: Regulating the Synthetic Society: Generative AI, Legal Questions, and Societal Challenges. Bloomsbury (2024)
Véliz, C.: Privacy is Power: Why and How You Should Take Back Control of Your Data. Transworld Publishes (2020)
Weidinger, L., Rauh, M., Marchal, N., Manzini, A., Hendricks, L.A., Mateos-Garcia, J., Bergman, S., Kay, J., Griffin, C., Bariach, B., Gabriel, I., Rieser, V., Isaac, W.: Sociotechnical Safety Evaluation of Generative AI Systems. In arXiv (2023). https://doi.org/10.48550/arXiv.2310.11986)
Wong, P.-H., Wang, T.X. (eds.): Harmonious Technology: A Confucian Ethics of Technology. Routledge (2021)
Wu, X., Duan, R., Ni, J.: Unveiling Security, Privacy, and Ethical Concerns of ChatGPT (2023). https://doi.org/10.48550/arXiv.2307.14192). In arXiv
Zhou, J., Müller, H., Holzinger, A., Chen, F.: Ethical ChatGPT: Concerns, Challenges, and Commandments (2023). https://doi.org/10.48550/arXiv.2305.10646). In arXiv
Zhuo, T.Y., Huang, Y., Chen, C., Xing, Z.: Red teaming ChatGPT via Jailbreaking: Bias, Robustness, Reliability and Toxicity (2023). https://doi.org/10.48550/arXiv.2301.12867). In arXiv
Author information
Authors and Affiliations
Corresponding author
Ethics declarations
Competing interests
The authors declare that they have no competing interests in relation to the current paper.
Additional information
Publisher’s note
Springer Nature remains neutral with regard to jurisdictional claims in published maps and institutional affiliations.
Rights and permissions
Open Access This article is licensed under a Creative Commons Attribution-NonCommercial-NoDerivatives 4.0 International License, which permits any non-commercial use, sharing, distribution and reproduction in any medium or format, as long as you give appropriate credit to the original author(s) and the source, provide a link to the Creative Commons licence, and indicate if you modified the licensed material. You do not have permission under this licence to share adapted material derived from this article or parts of it. The images or other third party material in this article are included in the article’s Creative Commons licence, unless indicated otherwise in a credit line to the material. If material is not included in the article’s Creative Commons licence and your intended use is not permitted by statutory regulation or exceeds the permitted use, you will need to obtain permission directly from the copyright holder. To view a copy of this licence, visit http://creativecommons.org/licenses/by-nc-nd/4.0/.
About this article
Cite this article
Steen, M., Greeff, J., Boer, M. et al. Ethical aspects of ChatGPT: An approach to discuss and evaluate key requirements from different ethical perspectives. AI Ethics (2024). https://doi.org/10.1007/s43681-024-00571-x
Received:
Accepted:
Published:
DOI: https://doi.org/10.1007/s43681-024-00571-x