Abstract
In this retrospective observational study, we aimed to develop a machine-learning model using data obtained at the prehospital stage to predict in-hospital cardiac arrest in the emergency department (ED) of patients transferred via emergency medical services. The dataset was constructed by attaching the prehospital information from the National Fire Agency and hospital factors to data from the National Emergency Department Information System. Machine-learning models were developed using patient variables, with and without hospital factors. We validated model performance and used the SHapley Additive exPlanation model interpretation. In-hospital cardiac arrest occurred in 5431 of the 1,350,693 patients (0.4%). The extreme gradient boosting model showed the best performance with area under receiver operating curve of 0.9267 when incorporating the hospital factor. Oxygen supply, age, oxygen saturation, systolic blood pressure, the number of ED beds, ED occupancy, and pulse rate were the most influential variables, in that order. ED occupancy and in-hospital cardiac arrest occurrence were positively correlated, and the impact of ED occupancy appeared greater in small hospitals. The machine-learning predictive model using the integrated information acquired in the prehospital stage effectively predicted in-hospital cardiac arrest in the ED and can contribute to the efficient operation of emergency medical systems.
Similar content being viewed by others
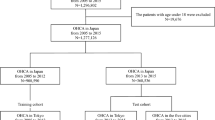
Explore related subjects
Discover the latest articles, news and stories from top researchers in related subjects.Introduction
Critically ill patients usually arrive at the emergency department (ED) through emergency medical services (EMS). Paramedics must quickly recognize the patient’s condition, and transport them to the optimal hospital, while providing appropriate first aid. Several studies have been conducted to develop a prehospital prediction tool for differentiating general patients from patients who are critically ill or have specific conditions, such as myocardial infarction or stroke, that require immediate treatment1,2,3,4. Since the ED and EMS are increasingly overloaded, and ambulance diversion is frequent, it is important for paramedics to accurately select and transport critically ill patients to the appropriate hospital to save their lives and support the efficient operation of medical facilities5,6,7.
With the growing demand for high-quality healthcare, embedding artificial intelligence (AI) into healthcare systems is a solution which promises to improve productivity and efficiency8,9. Since in-hospital cardiac arrest (IHCA) has a low survival rate, and is a major public healthcare burden that causes intensive consumption of medical resources, it is valuable to predict and minimize the occurrence of IHCA10,11. Recently, several studies that used AI to predict IHCA through patient clinical features have reported that AI-based models are superior to conventional rule-based tools12,13,14. However, to our knowledge, no studies have been conducted to predict the occurrence of IHCA at the prehospital stage. Because it can be challenging to assess information and make accurate and objective decisions in chaotic prehospital scenes, automated AI predictive models can be used to help paramedics make optimal decisions. In addition, the quality of care in the ED is adversely affected by crowding and overwhelmed medical staff. Previous studies have also reported that ED crowding increases the incidence of IHCA10,15. Therefore, the patient’s clinical features and hospital conditions should be considered to predict IHCA occurrence. AI can be an effective tool to play an auxiliary role in decision-making by integrating such a wide range of information.
In this study, we developed a machine-learning (ML) model to predict the occurrence of IHCA in the ED of patients arriving via EMS. Our research hypothesis was that an ML model that includes both patient clinical data and hospital factors could effectively predict the occurrence of IHCA at the prehospital stage.
Methods
Study design and setting
We conducted a retrospective, observational cohort study using a nationwide dataset that matched the National Fire Agency (NFA) data to the National Emergency Department Information System (NEDIS) in Korea. The Korean NFA is responsible for responding to fire, disaster, rescue, and EMS, following the Framework Act on Fire Services. The NFA has service headquarters in 18 cities and provinces and operates 210 fire stations. When paramedics transfer an emergency patient from the scene to the ED, they fill out a transfer record using a nationwide unified form and submit it to the NFA. The transfer record is written and transmitted in electronic form through a device owned by paramedics and managed by the NFA. Since 2013, the transfer record by paramedics has been amended four times by the Expert Quality Management Committee. This committee conducts audit and quality control of the NFA data registry and operates a data-based prehospital quality management program.
Hospitals must transmit emergency patient information via the NEDIS, a computerized system that collects data, such as clinical information and treatment results, of patients visiting ED nationwide. This system is managed by the National Emergency Medical Center (NEMC). Hospitals are also obligated to periodically transmit data related to ED crowding, such as the number of beds and occupants. This crowding information is disclosed online in real time for public purposes. Our study data were constructed by attaching the NFA’s prehospital information and ED crowding status at the time of patient arrival to the data of each patient registered in the NEDIS.
This study included patients transported to the ED by EMS and had information transmitted to the NEDIS from September 2017 to December 2018. Children younger than 18 years of age, and those who experienced cardiac arrest before ED arrival, were excluded from the study. Data were provided anonymously by the NFA and NEMC, and the work was performed in a secure manner on a computer that only the researchers in this study had access to. The institutional review boards of Severance Hospital permitted us to proceed with the study, including an exemption from obtaining the patient’s informed consent (4-2021-0580). This research was conducted in accordance with the principles in the Declaration of Helsinki.
Data processing
The NEMC recommends that crowding data be automatically transmitted at least once every 15 min or at least once every hour for manual transmissions. We obtained NEDIS data for our sample, including the patients’ age and sex, arrival time and treatment codes at the ED. The number of hospitals, beds, and patients occupying the ED at arrival was added to the matched patient data. We selected the crowding data transmitted closest to the patient’s arrival at the ED.
The NFA data included the following variables: patient’s age, sex, category of non-medical problems (e.g. hanging, trauma, or poisoning), medical history, symptoms, level of consciousness, vital signs (blood pressure, pulse rate, body temperature, and oxygen saturation), blood sugar level, emergency care provided during transport (cardiopulmonary resuscitation (CPR), laryngeal mask airway, oxygen administration, and intravenous infusion of fluid), phone call time, ED arrival time, area of occurrence, and the name of the hospital to which the patient was transferred. Hypo- and hyperglycemia were defined as blood sugar levels below 80 mg/dL and above 250 mg/dL, respectively.
We matched the NFA data to the NEDIS data based on patient age, sex and arrival time. We accepted a 10-min difference for the arrival time of the two datasets. If two or more patients in the NFA data were matched for one patient in the NEDIS database, the records were matched to the hospital name.
Since the NEDIS data contained information on the treatment performed in the ED, we used it to determine the occurrence of sudden IHCA requiring resuscitation. The electronic data interchange code M1583-7, corresponding to CPR, was used to define the IHCA group. Patients were excluded if the NFA data indicated CPR before arrival at the hospital. ED occupancy rate was used as an indicator of crowding. We calculated the occupancy rate by dividing the number of occupants by the number of beds16. Although there are no globally agreed representative indicator for measuring ED crowding, the occupancy rate is one of the most promising methods for quantification17.
Model development
The complete dataset was randomly split into training and test sets in an 8:2 ratio. We used the training set to develop the prediction model for IHCA. Two datasets were generated: one containing all variables, including hospital factors, and a second model containing only patient factors (excluding hospital factors). We trained three ML models: logistic regression (LR), extreme gradient boosting (XGB, XGBoost), and multilayer perceptron network (MLP). The training set was split into 10 folds. We performed grid-search for hyperparameter tuning and the details of search space and selected setting are in Supplementary Table 1. In order to resolve the data imbalance problem, we applied naïve random sampling in training folds where the validation fold and test set were untouched. First, we oversampled IHCA positive samples to raise the class ratio by 10%. Next, we under-sampled negative samples to change the class ratio by 30%. After random sampling, the size of training folds was shrunk to 30% of the original total training folds. The area under the receiver operating characteristic curve (AUROC) and area under the precision-recall curve (AUPRC) were calculated in the test fold in every epoch. We used the highest AUROC score among every ten iterations to select the single best model from LR, XGB, and MLP.
Model validation
The performances of the LR, XGB and MLP models with and without hospital factors were validated in the test set. Model performance was estimated using AUROC, AUPRC, sensitivity, specificity, accuracy, positive predictive value (PPV), and negative predictive value (NPV) with 95% confidence intervals. We compared the AUROC values of different model algorithms, and selected the final model with the highest AUROC value.
In order to interpret the final predictive model, we adopted the SHapley Additive exPlanation (SHAP) proposed by Lundberg and Lee in 201718. SHAP can explain any ML model’s output by calculating the impact of each feature on model prediction based on game theory. SHAP allows us to understand which feature is the most important to model prediction, and the positive or negative direction of the feature impact. Applying SHAP to our developed model, we used the DeepExplainer module, which enables the fast approximation of SHAP values in the deep learning model, and the TreeExplainer module, which is an optimized SHAP algorithm for tree ensemble methods such as XGB19. First, we ranked the feature importance by SHAP values. We calculated the AUROC score in the test set, starting from feature values all set by zero-value, sequentially adding one feature to replace feature values from zero to their own data. We confirmed the increasing trend of AUROC when variables were added one by one in the order of the most influential variables.
Considering that the patient characteristics and frequency of IHCA could differ depending on the size of the hospital, additional validation was carried out in four subgroups defined by the quartile of the number of hospital beds. We confirmed the SHAP value of ED crowding according to hospital size by visualizing the dependence plot to evaluate the impact of the hospital factors.
Statistical analysis
Continuous variables were presented as the mean and standard deviation and compared between groups using Student’s t test. Nominal variables are expressed in frequency and fraction and analyzed using the Chi-square test. We developed models using three ML methods, LR, XGB and MLP, and compared the AUROC of each model using the DeLong test to determine whether the difference between models was statistically significant20. Meanwhile, the significance of the AUPRC difference was calculated by a bootstrap of 1000 iterations. Estimations of confidence intervals by single model prediction were obtained from Hanley JA for AUROC, Boyd for AUPRC, Wilson score interval for sensitivity, specificity, and accuracy, and Mercaldo ND for PPV and NPV21,22,23,24. The optimal cut-off value was calculated using Jouden’s index. We calibrated the final model by platt scaling and isotonic regression and checked the Brier score. All statistical analyses were implemented and performed in Python with the SciPy and scikit-learn packages, and p-values < 0.05 were considered statistically significant.
Results
We identified 1,530,160 patients from the NEDIS who arrived nationwide at the ED via EMS during the study period (Fig. 1). Within that group, 23,504 patients did not match the NFA data. Among 1,506,656 patients who succeeded in matching, we excluded 120,465 patients under 18, 27,560 patients with out-of-hospital cardiac arrest, and 7938 patients with missing data. Our final data set consisted of 1,350,693 eligible patients. The training set had 1,080,554 individuals, and the test set consisted of 270,139 individuals. The clinical characteristics of the patients in the training and test sets were similar and are presented in Supplementary Table 2. IHCA occurred in 5431 patients (0.4%). Patients in the IHCA group were significantly older (70.13 ± 15.50 vs. 57.98 ± 19.09, p < 0.001) and had a lower proportion of men than those in the non-IHCA group (Table 1). The IHCA group had a higher mean number of hospital beds (761.65 ± 462.17 vs. 621.97 ± 441.80, p < 0.001) and higher ED occupancy (0.63 ± 0.51 vs. 0.45 ± 0.36, p < 0.001).
Model validation
The performances of the models were evaluated using a test set, and ML models with a combination of naïve random over/under-sampling did not show better performance (Supplementary Table 3). Figure 2 shows the ROC and PR curves of models predicting the occurrence of IHCA developed using LR, XGB, and MLP. The AUROC of all three ML models showed high performance with 0.9 or higher, and XGB, including the hospital factor, was the highest at 0.9267. In AUPRC, the value of the XGB model, including all variables, was 0.1319, the highest compared with LR and MLP. The comparative analysis of AUROC and AUPRC between the models are shown in Supplementary Table 4. We used logistic regression and isotonic regression for probability calibration and the calibrators were fit with validation fold. We selected the ML model of the XGB method, including all variables, as the final model. The Brier score of the final XGB model was 0.0037 and the calibration plot is shown in Supplementary Fig. 1.
The performance of the final model was verified using four subgroups of the test set. The test set was divided into quartiles (Q1–Q4) bordering 296, 568, and 818 of the number of hospital beds. From Q1 to Q4, there were more patients with dyspnea, chest pain, and mental change, and more emergency care was performed at the site. The incidence of IHCA in the ED was also higher (Supplementary Table 5). The AUROC of the model’s prediction of IHCA occurrence was evaluated to be 0.9 or higher in all subgroups, and the AUPRC increased from Q1 to Q4 and was the highest at 0.1532 in Q4 (Supplementary Fig. 2). Supplementary Table 6 presents the performance analysis results of the subgroups.
Model interpretation
The parsimony plot in Fig. 3 shows the trend of increasing the AUROC of the final model when variables were added one by one in the top-ranking order. The largest increase in model performance was observed from the first to the eighth variables, after which marginal gains in performance were added as the remaining variables were input. The most influential variable was oxygen supply, followed by age, oxygen saturation, and systolic blood pressure. The number of ED beds and ED occupancy were the fifth and sixth most influential variables in the model performance, respectively.
Figure 4 shows the SHAP of the variables when the model was applied to the test set. The top ten variables with the most influence on the model are shown on the y-axis in rank order. As shown in the parsimony plot, oxygen supply, age, oxygen saturation, and systolic blood pressure were the top four most influential variables in the test set, which was also confirmed in the subgroups. ED occupancy was selected as the 5th or 6th most influential variable, and ED occupancy and IHCA occurrence were positively correlated in large and small hospitals. Figure 5 shows a dependence plot of the SHAP value of ED occupancy according to the number of hospital beds. Compared with hospitals with many beds, the SHAP value of ED occupancy tends to be higher in small hospitals.
Discussion
In this study, we found that the ML-based predictive model using integrated information acquired in the prehospital stage effectively predicted the occurrence of IHCA in the ED. We also confirmed that hospital factors such as ED crowding, were the main factors in the prediction model. The application of ML for decision-making is gradually expanding in the medical field, including during the prehospital stage25,26. However, while the data generated during the prehospital stage are not yet fully utilized in ML research, it is significant that we developed a predictive model with integrated information based on the national dataset extracted from the standardized prehospital care system.
All three machine-learning algorithms trained in our study showed AUROC values above 0.9 for ED IHCA prediction. The disadvantage of the imbalanced dataset is that the AUROC is optimistic; therefore, all our models yielded high AUROC results, which were statistically significant but with minimal numerical differences. Therefore, it is desirable to consider AUPRC along with AUROC for model performance. AUPRC was 0.13 in XGB, which was higher than that of LR and MLP. The AUPRC is a single number summary of the information in the precision-recall curve, and is a useful performance metric for imbalanced data in a setting focused on finding positive examples24,27. While the baseline of AUROC is always 0.5, AUPRC is a relative indicator because the baseline is the proportion of positive cases to the population24. In our dataset, which has 0.004 as the baseline AUPRC, an AUPRC value of 0.132 by the XGB algorithm reflects favorable performance in IHCA prediction. XGB, a tree ensemble model, has been reported to perform better in classification and regression problems involving tabular data organized in rows and columns, which are the most common data types in traditional statistical modeling28,29,30.
The disadvantage of our model was that the AUPRC and PPV were very low due to the imbalanced dataset. Therefore, when applied to the real world, the problem of false alarms is inevitable. However, we expect that our predictive model has high sensitivity and can be used for the purpose of screening patients with the potential to develop IHCA. IHCA in the ED is sudden and unpredictable, but considered to be preventable if early identification of at-risk patients and adequate interventions are possible15,31. Therefore, a predictive model for screening patients at the emergency scene and transporting them to the optimal hospital will help reduce the incidence of IHCA and increase the survival of patients.
Machine learning-based predictive tools may raise doubts about their clinical applicability because they do not provide explanations supported by clinical relevance9,13. In this study, to overcome the black-box aspect of machine learning, we tried to present clinical validity by using an explanatory machine learning called SHAP. Based on the SHAP analysis, we found that clinical variables associated with the occurrence of IHCA (i.e. oxygen supply and saturation, vital signs, and mental status) in previous studies were in line with variables that had a high influence on the output of the present model32,33,34. In addition to these key factors, our model included more clinical data such as medical history, symptoms, first aid, and hospital factors, increasing the predictive power over traditional statistical methods. Making the optimal choice from such a wide range of information is an advantage of AI. However, these various data must be input in real time to apply AI models in an emergency scene, which cannot be done using a conventional manual system. Automated collection and rapid processing of real-time integrated data must be available to ensure the feasibility of predictive models.
Along with variables reflecting the patient’s medical condition, hospital factors such as the number of ED beds and ED occupancy also ranked highly as significant predictors of IHCA. These results were found consistently across all subgroups divided based on hospital size. A comparison of the patient characteristics of the subgroups showed that large hospitals have numerous ED beds, more crowded ED, and a higher frequency of IHCA. In the dependence plot analyzed to consider the relationship between these variables, we found a positive correlation between ED crowding and its impact on IHCA incidence in hospitals of all sizes. ED crowding is associated with a lack of resources for patients needing immediate resuscitation35. Previous studies have reported that ED crowding is associated with the occurrence of IHCA10,15. In the dependence plot, we could also confirm that ED crowding had a greater effect on the occurrence of IHCA in small hospitals than in large hospitals. In the case of ED crowding, small hospitals do not have sufficient medical resources available and are less able to cope with crises than large hospitals. For this reason, paramedics usually decide to transfer seriously ill patients in urgent situations to a larger hospital, but the level of crowding is often not considered. Although the ED crowding status is shared online, it is not easy for paramedics to manually search for this information in emergencies. As ED crowding is a factor that affects the quality of care, it is necessary to establish a process that can be considered for the selection of an appropriate transfer hospital by quickly obtaining crowding information when transporting emergency patients. In addition, because ED crowding causes ambulance diversion, paramedics can use this process to reduce the retransfer of emergent patients36.
Our study had several limitations. First, our study included the potential bias of its retrospective design. Second, as this study was conducted in a single country, caution is needed when generalizing the study results. Finally, the present predictive model has not been validated in the real world. In particular, since our predictive model has suboptimal performance metrics due to imbalanced data, the usefulness of the developed model needs to be verified through additional prospective studies before being applied to prehospital care. Establishing a digital platform to deploy the ML algorithm developed in this study is necessary to demonstrate its usefulness prospectively.
Conclusions
The ML-based predictive model developed by integrating various data, including hospital factors and patients’ clinical information generated in the prehospital stage, effectively predicted the occurrence of IHCA. The AI decision support system will enable evidence-based judgment with increased utilization of the information available in emergencies, thereby providing the basis for the efficient use of emergency medical resources while ensuring patient safety.
Data availability
The data that support the findings of this study are available from the National Fire Agency (NFA) and the National Emergency Medical Center (NEMC) but restrictions apply to the availability of these data, which were used under license for the current study, and so are not publicly available. Data are however available from the authors upon reasonable request and with permission of NFA and NEMC.
Abbreviations
- AI:
-
Artificial intelligence
- AUROC:
-
Area under the receiver operating characteristic curve
- AUPRC:
-
Area under the precision-recall curve
- CPR:
-
Cardiopulmonary resuscitation
- ED:
-
Emergency department
- EMS:
-
Emergency medical services
- IHCA:
-
In-hospital cardiac arrest
- LR:
-
Logistic regression
- ML:
-
Machine learning
- MLP:
-
Multilayer perceptron network
- NEDIS:
-
National Emergency Department Information System
- NEMC:
-
National Emergency Medical Center
- NFA:
-
National Fire Agency
- NPV:
-
Negative predictive value
- PPV:
-
Positive predictive value
- SHAP:
-
SHapley Additive exPlanation
- XGB:
-
Extreme gradient boosting
References
Seymour, C. W. et al. Prediction of critical illness during out-of-hospital emergency care. JAMA 304, 747–754 (2010).
Zhelev, Z., Walker, G., Henschke, N., Fridhandler, J. & Yip, S. Prehospital stroke scales as screening tools for early identification of stroke and transient ischemic attack. Cochrane Database Syst. Rev. 4, Cd011427. https://doi.org/10.1002/14651858.CD011427.pub2 (2019).
Sagel, D. et al. Prehospital risk stratification in patients with chest pain. Emerg. Med. J. 38, 814–819 (2021).
Silcock, D. J., Corfield, A. R., Gowens, P. A. & Rooney, K. D. Validation of the national early warning score in the prehospital setting. Resuscitation 89, 31–35 (2015).
Andrew, E., Nehme, Z., Cameron, P. & Smith, K. Drivers of increasing emergency ambulance demand. Prehosp. Emerg. Care 24, 385. https://doi.org/10.1080/10903127.2019.1635670 (2020).
Paulin, J., Kurola, J., Koivisto, M. & Iirola, T. EMS non-conveyance: A safe practice to decrease ED crowding or a threat to patient safety?. BMC Emerg. Med. 21, 115. https://doi.org/10.1186/s12873-021-00508-1 (2021).
Cooney, D. R. et al. Ambulance diversion and emergency department offload delay: Resource document for the national association of EMS physicians position statement. Prehosp. Emerg. Care 15, 555–561 (2011).
Taylor, R. A. & Haimovich, A. D. Machine learning in emergency medicine: Keys to future success. Acad. Emerg. Med. 28, 263–267 (2021).
Deo, R. C. Machine learning in medicine. Circulation 132, 1920–1930 (2015).
Chang, Y. H. et al. Association of sudden in-hospital cardiac arrest with emergency department crowding. Resuscitation 138, 106–109 (2019).
Nolan, J. P. et al. Incidence and outcome of in-hospital cardiac arrest in the United Kingdom national cardiac arrest audit. Resuscitation 85, 987–992 (2014).
Cho, K. J. et al. Detecting patient deterioration using artificial intelligence in a rapid response system. Crit. Care Med. 48, e285–e289. https://doi.org/10.1097/CCM.0000000000004236 (2020).
Hong, S., Lee, S., Lee, J., Cha, W. C. & Kim, K. Prediction of cardiac arrest in the emergency department based on machine learning and sequential characteristics: Model development and retrospective clinical validation study. JMIR Med. Inform. 8, e15932. https://doi.org/10.2196/15932 (2020).
Kwon, J. M., Lee, Y., Lee, Y., Lee, S. & Park, J. An algorithm based on deep learning for predicting in-hospital cardiac arrest. J. Am. Heart Assoc. 7, e008678. https://doi.org/10.1161/JAHA.118.008678 (2018).
Kim, J. S. et al. Maximum emergency department overcrowding is correlated with occurrence of unexpected cardiac arrest. Crit. Care 24, 305. https://doi.org/10.1186/s13054-020-03019-w (2020).
McCarthy, M. L. et al. The emergency department occupancy rate: A simple measure of emergency department crowding?. Ann. Emerg. Med. 51, 15–24 (2008).
Peltan, I. D. et al. Emergency department crowding is associated with delayed antibiotics for sepsis. Ann. Emerg. Med. 73, 345–355 (2019).
Lundberg, S. & Lee, S. A unified approach to interpreting model predictions. Preprint at https://arxiv.org/abs/1705.07874 (2017).
Lundberg, S. M. et al. From local explanations to global understanding with explainable AI for trees. Nat. Mach. Intell. 2, 56–67 (2020).
DeLong, E. R., DeLong, D. M. & Clarke-Pearson, D. L. Comparing the areas under two or more correlated receiver operating characteristic curves: A nonparametric approach. Biometrics 44, 837–845 (1988).
Mercaldo, N., Lau, K. & Zhou, X. Confidence intervals for predictive values with an emphasis to case-control study. Stat. Med. 26, 2170–2183 (2007).
Hanley, J. A. & McNeil, B. J. The meaning and use of the area under a receiver operating characteristic (ROC) curve. Radiology 143, 29–36 (1982).
Wilson, E. B. Probable inference, the law of succession and statistical inference. J. Am. Stat. Assoc. 22, 209–212 (1927).
Boyd, K., Eng, K. H. & Page, C. D. Area under the precision recall curve: Point estimates and confidence intervals. In Machine Learning and Knowledge Discovery in Databases (eds Blockeel, H. et al.) 451–466 (Springer Berlin Heidelberg, 2013). https://doi.org/10.1007/978-3-642-40994-3_55.
Spangler, D., Hermansson, T., Smekal, D. & Blomberg, H. A validation of machine learning-based risk scores in the prehospital setting. PLoS ONE 14, e0226518. https://doi.org/10.1371/journal.pone.0226518 (2019).
Pirneskoski, J. et al. Random forest machine learning method outperforms prehospital national early warning score for predicting one-day mortality: A retrospective study. Resusc. Plus 4, 100046. https://doi.org/10.1016/j.resplu.2020.100046 (2020).
Saito, T. & Rehmsmeier, M. The precision-recall plot is more informative than the ROC plot when evaluating binary classifiers on imbalanced datasets. PLoS ONE 10, e0118432. https://doi.org/10.1371/journal.pone.0118432 (2015).
Shwartz-Ziv, R. & Armon, A. Tabular data: Deep learning is not all you need. Inf. Fusion 81, 84–90 (2022).
Chen, T. & Guestrin, C. Xgboost: A scalable tree boosting system. In Proc. of the 22nd acm sigkdd International Conference on Knowledge Discovery and Data Mining 785-794 https://doi.org/10.1145/2939672.2939785 (2016).
Prokhorenkova, L., Gusev, G., Vorobev, A., Dorogush, A. V. & Gulin, A. CatBoost: Unbiased boosting with categorical features. Adv. Neural Inf. Process. Syst. https://doi.org/10.5555/3327757.3327770 (2018).
Hoybye, M. et al. In-hospital vs. out-of-hospital cardiac arrest: Patient characteristics and survival. Resuscitation 158, 157–165 (2021).
Tsai, C. L. et al. Development and validation of a novel triage tool for predicting cardiac arrest in the emergency department. West. J. Emerg. Med. 23, 258–267 (2022).
Acharya, P. et al. Incidence, predictors, and outcomes of in-hospital cardiac arrest in COVID-19 patients admitted to intensive and non-intensive care units: Insights from the AHA COVID-19 CVD registry. J. Am. Heart. Assoc. 10, e021204. https://doi.org/10.1161/JAHA.120.021204 (2021).
Srivilaithon, W. et al. Predictors of in-hospital cardiac arrest within 24 h after emergency department triage: A case-control study in urban Thailand. Emerg. Med. Australas. 31, 843–850 (2019).
Hong, K. J., Shin, S. D., Song, K. J., Cha, W. C. & Cho, J. S. Association between ED crowding and delay in resuscitation effort. Am. J. Emerg. Med. 31, 509–515 (2013).
Castillo, E. M. et al. Collaborative to decrease ambulance diversion: The California emergency department diversion project. J. Emerg. Med. 40, 300–307 (2011).
Acknowledgements
This study was supported by a new faculty research seed money grant of Yonsei University College of Medicine for 2021 (6-2021-0140) and a grant from the 2019 IT Promotion fund (Development of AI based Precision Medicine Emergency system) of the government of Korea (Ministry of Science and ICT) (Grant Number: S1015-19-1001).
Author information
Authors and Affiliations
Contributions
J.H.K.: Conceptualization, methodology, writing – original draft, A.C.: Conceptualization, methodology, writing – review & editing, M.J.K.: Conceptualization, writing – original draft, writing – review & editing, data curation, supervision, H.H.: Formal analysis, visualization, S.K.: Data curation, formal analysis, H.J.C.: Supervision.
Corresponding author
Ethics declarations
Competing interests
The authors declare no competing interests.
Additional information
Publisher's note
Springer Nature remains neutral with regard to jurisdictional claims in published maps and institutional affiliations.
Supplementary Information
Rights and permissions
Open Access This article is licensed under a Creative Commons Attribution 4.0 International License, which permits use, sharing, adaptation, distribution and reproduction in any medium or format, as long as you give appropriate credit to the original author(s) and the source, provide a link to the Creative Commons licence, and indicate if changes were made. The images or other third party material in this article are included in the article's Creative Commons licence, unless indicated otherwise in a credit line to the material. If material is not included in the article's Creative Commons licence and your intended use is not permitted by statutory regulation or exceeds the permitted use, you will need to obtain permission directly from the copyright holder. To view a copy of this licence, visit http://creativecommons.org/licenses/by/4.0/.
About this article
Cite this article
Kim, J.H., Choi, A., Kim, M.J. et al. Development of a machine-learning algorithm to predict in-hospital cardiac arrest for emergency department patients using a nationwide database. Sci Rep 12, 21797 (2022). https://doi.org/10.1038/s41598-022-26167-1
Received:
Accepted:
Published:
DOI: https://doi.org/10.1038/s41598-022-26167-1
- Springer Nature Limited
This article is cited by
-
Selection of consistent breath biomarkers of abnormal liver function using feature selection: a pilot study
Health and Technology (2023)